Abstract
Content
- Introduction
- 1. The general formulation of the problem
- 2. Research and selection methods
- 2.1 The method of cumulative frequency
- 2.2 The method of Shannon
- 2.3 The method of Kullback
- 2.4 The analysis method
- Conclusion
- References
Introduction
Cardiovascular disease (CVD) are the leading cause of death worldwide. It is estimated that 2008 deaths from CVD 17.3 million, representing 30% of all deaths in the world. Of these, 7.3 million people died of coronary heart disease and 6.2 million due to stroke.
Perhaps the most important goal of medical research is the classification of the object or in relation to the patient and the disease is diagnosis. The diagnosis can be formulated as a mathematical problem, and therefore automatic.
1. The general formulation of the problem
The main objective is to develop problem-oriented statistical analysis of biomedical information (the symptoms) of patients with CVD in order to predict or prevent the risk of the disease diagnosed patient. Under the definition of informative analysis of mean trait, which means, as far as this character describes the psycho-physical state of the object (patient), that is, how much of this feature depends on the diagnosis.
To confirm the relevance of the designed SCS, consider some of the features currently available to assess the achievements of information content:
- the majority of techniques developed for specific diseases, and often unfit for others;
- analysis of the data is by statistical methods, and most of the conclusions of statistical studies done under the condition of normality of data distributions, which is not true for all biomedical indicators;
- not well understood the importance of many factors that influence the diagnosis, and often in the studies examined only those features that are thought most clearly reflect the disease;
- due to the complexity of the data does not always apply the most powerful tests and doctors is limited, for example, a linear approximation or the power equation.
Approximate structure of the SCS is shown in Figure 1. The input data of SCS will be the database of the Donetsk hospital occupational diseases. The structure of the SCS will include all of the blocks below the block "Database". The main block of the designed SCS is a "processing unit". This unit is supposed to perform the sample characteristics with the subsequent calculation of their information.
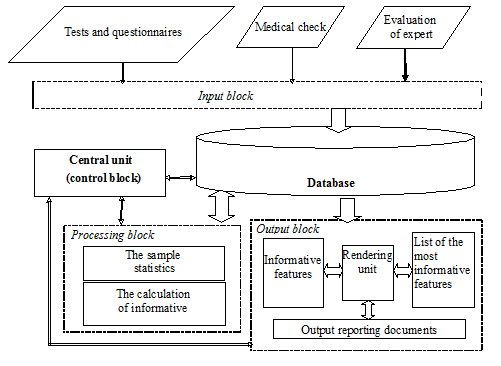
Figure 1 – The structure of the SCS
2. Research and selection methods
There are at least two approaches to evaluating information content is energy and information. The energy approach is based on the fact that information content is measured by the value of the trait.
The signs are ordered by size, and most informative is the one whose value is greater. For example, the amplitude-time analysis of ECG among the most informative feature is the amplitude of R wave amplitude.
However, such an approach to assessing the information content may be poorly suited to detect the object. Indeed, if any, sign of a large in absolute value, but almost the same for objects of different classes, then the value of this attribute is difficult to attribute to any object class.
And vice versa, if the sign is relatively small in magnitude, but very different from objects of different classes, then its value can easily be classified as an object.
Therefore more suitable for object recognition is the informational approach, under which the information criterion is considered as a significant difference between classes of images in the feature space.
If you object to recognize a need to refer to one of 2 classes, as such a significant difference may be a sign of the difference of probability distributions, constructed from samples from 2 classes being compared.
Assessment of informativeness is the value of I(xj) is area of a distribution of feature xj, is not shared with another area of distribution of the same sign.
2.1. The method of cumulative frequency
The essence of this method is that if there are two sample sign x, belonging to two different classes, then on both samples in the same coordinate axes construct the empirical distribution of the trait x and calculate cumulative frequency (the sum of the frequencies from the initial to the current range of distribution).
Assessment of informativeness is the modulus of the maximum difference between the cumulative frequency.
2.2. The method of Shannon
Shannon's method is evaluate offers informative as the weighted average number of information attributable to the different gradations of character. Under the information in the information theory to understand the value corrected for the entropy.
Thus, the information content of the j-th attribute:
![]() |
(1) |
G – gradation of character;
K – number of classes;
Pi – the probability of the i-th gradation characteristic.
![]() |
(2) |
mi,k – frequency of the i-th grades in the K-th class;
N – total number of observations.
Pi,k – the probability of the i-th feature in grades K class.
![]() |
(3) |
2.3. The method of Kullback
Another method for estimating the information content a method of Kullback is offers as a measure of informativeness measure the differences between the two classes, called divergence [1].
According to this method Kullback divergence, or information content is calculated by the formula:
![]() |
(4) |
G – number of G-gradation characteristic;
Pi1 – the probability of the i-th grades in first grade.
![]() |
(5) |
mi1 – frequency of occurrence of the i-th grades in the first class;
Denominator is the appearance of all grades in first grade, that is, the total number of observations in the first grade.
Pi2 – the probability of the i-th grades in the second grade.
![]() |
(6) |
mi2 – frequency of occurrence of the i-th grades in the second grade.
2.4 The analysis methods
To decide on the way to estimate the informativeness of a comparative analysis of three methods for determining the informativeness of the trait.
-
Dependence on the method of encoding methods trait.
The method of cumulative frequency (MCF) will depend on how the encoding attribute, the methods of Shannon and Kullback do not depend on the method of encoding. -
The dependence of the methods of the class number.
MCF and the method used to determine the Kullback informativeness criterion, which is involved in the recognition of only two classes of objects. The method allows to determine the Shannon information content of character involved in the recognition of an arbitrary number of classes of objects. -
The dependence of the number of methods of gradation characteristic.
All three methods do not depend on the number of shades of character. -
The dependence of the methods of sample size.
Since the MCF operates frequencies, then the sample size of observations should be the same sign on both recognizable classes. Methods of Kullback and Shannon operate probabilities, so the sample sizes of observations on two recognizable character classes can be different. -
The dependence of the methods of calculation.
MCF is easier in terms of computation. Methods of Kullback and Shannon is more complicated. -
The universality of the methods and the dependence on the magnitude of information content.
Information content, determined by all three methods is positive, but the MCF and the method of Kullback it is not normalized, so an informative, some of these methods can only speak in relative terms is higher or lower compared to other informative feature. The method gives an estimate of the Shannon information content as a normalized value, which varies from 0 to 1. therefore, an informative character, defined by Shannon is possible to speak in absolute terms: the closer to 1 – high, close to the 0 – low.
Method for determination of information content chosen by the researcher, depending on the objectives of the study, the number of recognized classes, medical and biological data is a method of encoding, the sample size the number of gradations.
Whichever way is used, if the information content to evaluate all the evidence in the same way, we can choose a more informative and less informative drop characteristics for the formulation of a specific diagnosis.
Conclusion
Based on the goals and objectives developed by SCS structure is formed. The methods of implementation of the basic problem – estimates of informative. According to the results of comparative analysis of selected method and algorithm of its realization. Modified database structure that will allow to observe the dynamics of the functional state of the patient on the minimum number of indicators.
In writing this essay master's work is not yet complete. Final completion: December 2012. The full text of the work and materials on the topic can be obtained from the author or his head after that date.
References
- Айвазян, С. А. Классификация многомерных наблюдений / С. А. Айвазян, З. И. Бежаева, О. В. Староверов. – М. : Статистика, 1974. – 200 с.
- Аркадьев А. Г. Обучение машины классификации объектов / А. Г. Аркадьев, Э. М. Браверманн. – М. : Наука, 1971. – 172 с.
- Генкин А. А. Новая информационная технология анализа медицинских данных; Программный комплекс ОМИС / А. А. Генкин. – СПб. : Политехника, 1999. – 191 с.
- Гублер Е. В. Алгоритм оценки расхождения распределений признаков в медицинских автоматизированных системах // Проблемы системотехники и автоматизированные системы управления. / Е. В. Гублер. – Л. : Медицина, 1978. – 230 с.
- Гублер Е. В. Вычислительные методы анализа и распознавания патологических процессов / Е. В. Гублер. – Л. : Медицина, 1978. – 296 с.
- Гублер Е. В. Применение непараметрических критериев статистики в медико-биологических исследованиях / Е. В. Гублер, А. А. Генкин – Л. : Медицина, 1973. – 144 с.
- Давнис В. В. Прогнозные модели экспертных предпочтений: монография / В.В. Давнис, В.И. Тинякова. – Воронеж: Изд-во Воронеж. гос. ун-та, 2005. – 248 с.
- Евтушенко Г. С. Выбор информативных признаков. Оценка информативности / Евтушенко Г.С.// Методические указания к лабораторной работе по дисциплине «Методы обработки биомедицинских данных» для бакалавров по направлению 553400 «Биомедицинская инженерия». – Томск: Изд. ТПУ, 2003. – 18 с. [Электронный ресурс] – Режим доступа: http://ime.tpu.ru/study....
- Ивантер Э. В. Основы биометрии: введение в статистический анализ биологических явлений и процессов: учебное пособие / Э. В. Ивантер, А. В. Коросов. – Петрозаводск: ПГУ, 1992. – 163 с.
- Сердечно-сосудистые заболевания / Центр СМИ // Информационный бюллетень. – 2011. – Сентябрь. – №17. [Электронный ресурс] – Режим доступа: http://www.who.int/mediacentre....