Abstract
Content
- 1. Objectives
- 2. The relevance of the topic
- 3. Prospective scientific novelty
- 4. Planned practical results
- 5. Development agent system competency-based learning automata models
- 5.1 Organization of agent-based subsystems learner competency assessment
- 6. Findings
- 7. List of sources
Objectives
The aim is to develop and study of automatic learning models based on analysis of learner competencies and development of additional multi-agent learning.
To achieve this goal requires the following tasks::
- Perform an analysis of the existing systems of training and testing, implementing training competencies
- Develop the composition and structure of the agent system of training and assessment of competence of trainees
- To formalize the process of training and testing based automata approach and the organization of multi-agent learning system
The object of this study are multi-agent systems competency training.
The subject of the study are automata models of learning and testing.
2. The relevance of the topic
In connection with the problems of modernization of the education system in higher education in the context of the Bologna Declaration is updated is the move to the planning of educational outcomes in language skills. The educational approach based on the designation of competence as a goal of training a specialist in high school, defined as competence. Application of the competency approach is the formation of individual programs of study relevant characteristics of each student [1], and addressing the needs of companies in the vocational guidance specialists. This can be implemented using distributed intelligent agent-oriented technologies that achieve the required competencies by the introduction of adaptability in the learning process. The main objective is to ensure the optimal trajectory to the goal of learning by constructing an individual learning plan and managed assimilation of educational material in the learning system.
The problems of determining the current competence [2] of the trainee and control the formation of competencies addressed in existing systems through the use of technology control test, when the subject is asked to perform pre-defined tasks of varying difficulty.
The disadvantage of the existing testing tools training systems is to focus primarily on the diagnosis and assessment of the quality of knowledge and skills acquired by the student as a result of the development of specific disciplines and practices. Testing the traditional tools do not allow to test and evaluate high, productive levels of knowledge.
Thus, for systems based learning competencies characterized by the use of adaptation on the levels of training and knowledge testing. Analysis of existing approaches to the implementation of controls training and testing shows that the automaton may be a promising approach to simplify the implementation of training scenarios and testing complex logic.
3. Prospective scientific novelty
Scientific novelty of the research is to develop new models of automatic intelligent [3] agents learning systems and algorithms for the implementation of competencies of individual trajectories of training and testing that improve the quality of education to meet modern needs of employers in vocational guidance specialists.
4. Planned practical results
The practical significance of the results depends on the choice of the priority directions of the development of intelligent tutoring systems with individual education programs [10]. Designed automata models and control algorithms for training and testing can be used in multi-agent systems, the new generation of distance learning-oriented training based on competencies.
5. Development agent system competency-based learning automata models
5.1 Organization of agent-based subsystems learner competency assessment
Intelligent multi-agent learning system (IMLS) is organized as a community of interacting groups of agents. The process of training and assessment of competence is provided by two groups (subsystems) agents. Communications teams of agents are shown in Figure 1. The collective of agents training (CLA) interacts with an agent trainee (AT) and the repository of learning resources (RLR). The collective of agents testing (CTA) is associated with AT and repository of test resources (RTR). Companies training and testing agents also interact with each other. The figure shows only the components IMLS relevant to the subsystem developed by CTA.
Dynamic learner profile stores information about the student, it records all received during training and proven competence test.
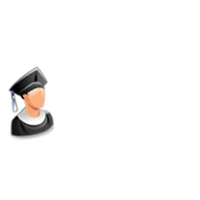
Figure 1 - Composition IMLS
Animation: 12 frames, 5 cycle of repetition, 52 KB
To better assess the knowledge to be used for the required competence ontology and on that basis to build customized learning paths and testing.
Findings
The proposed system can be used to enhance learning competency-based approach. The use of a repository of test resources will accumulate test items for the required competencies and based on them to build customized tests. Use of this system will automatically generate a sequence of educational material - the main program and re-training, according to the learner's current competence.
This master’s work is not completed yet. Final completion: December 2013. The full text of the work and materials on the topic can be obtained from the author or his scientific adviser after this date.
List of sources
1. Тельнов Ю.Ф. Управление компетенциями в самообучающейся организации / Ю.Ф. Тельнов // V-я Международная научно-практическая конференция «Интегрированные модели и мягкие вычисления в искусственном интеллекте», Коломна, 2009.
2. Carbonell J.R. AI in CAI: an Artificial Intelligence Approach to Computer-Aided Instruction // IEEE Transactions on Man-Machine Systems. – 1970. – Vol. MMS-11. – №4.
3. Стефанюк В.Л. Введение в интеллектуальные обучающие системы.учебно-методическое пособие / В.Л. Стефанюк. – М.: Росийский университет дружбы народов, – 2002. – 59 с.
4. Брусиловский П.Л. Интеллектуальные обучающие системы / П.Л. Брусиловский // Информатика. Информационные технологии. Средства и системы. – 1990. – № 2. – С. 3-22.
5. Петрушин В.А. Экспертно-обучающие системы / В.А. Петрушин. – Киев: Наукова думка, – 1992. – 352 с.
6. Tang, T.Y., Wu, A. The implementation of a multi-agent intelligent tutoring system for the learning of computer programming // Proceedings of 16th IFIP World Computer Congress-International Conference on Educational Uses of Communication and Information Technology. (ICEUT). 2000. pp. 56-67
7. Lopes F., Mamede N., Novais A. Q. and Coelho H. 2000. Towards a Generic Negotiation Model for Intentional Agents// In Proceedings of the IEEE Workshop on Agent-Based Information Systems (London, UK). IEEE Computer Society Press, CA, Р. 433-439.
8. Корнеев Д.Г., Тельнов Ю.Ф., Козлова О.А. МОДЕЛЬ ДИНАМИЧЕСКОЙ СИСТЕМЫ ФОРМИРОВАНИЯ КОМПЕТЕНЦИЙ НА ОСНОВЕ АГЕНТНО-ОРИЕНТИРОВАННОГО ПОДХОДА ИНФОРМАЦИОННО-КОММУНИКАЦИОННЫЕ ТЕХНОЛОГИИ В СФЕРЕ КУЛЬТУРЫ // Сборник научных трудов по итогам Международной научно-методической конференции (г. Саратов, 19-24 сентября 2011 г.) C. 14-16
9. Рыбина Г.В. Автоматизированное рабочее место для построения интегрированных экспертных систем: комплекс АТ-ТЕХНОЛОГИЯ / Г.В. Рыбинa // Новости искусственного интеллекта. 2005. № 3. С. 69-87.
10. Рыбина Г.В. РЕАЛИЗАЦИЯ КОМПЕТЕНТНОСТНОГО ПОДХОДА К ОБУЧЕНИЮ НА ОСНОВЕ РАЗРАБОТКИ ОБУЧАЮЩИХ ИНТЕГРИРОВАННЫХ ЭКСПЕРТНЫХ СИСТЕМ / Г.В. Рыбина // Научная сессия МИФИ-2008. Сборник научных трудов. Т.10: Интеллектуальные системы и технологии. М.: МИФИ, – 2008. – 41 c.
11. IMS Question and Test Interoperability Implementation Guide [Электронный ресурс]. – Режим доступа: http://www.imsglobal.org/question/qtiv2p1pd2/imsqti_implv2p1pd2.html - Загл. с экрана.
12. Система прохождения и создания тестов DiTest.v2 [Электронный ресурс]. – Режим доступа: http://ditest.edu.nstu.ru/ - Загл. с экрана.
13. «INDIGO» – программа для создания тестов и тестирования [Электронный ресурс]. – Режим доступа: http://indigotech.ru/ - Загл. с экрана.
14. Редактор электронных курсов CourseLab [Электронный ресурс]. – Режим доступа: http://websoft.ru/db/wb/root_id/ courselab/ doc.html - Загл. с экрана.
15. ФЕДОРУК П.І. ГРАФО-АВТОМАТНА МОДЕЛЬ АДАПТИВНОЇ СИСТЕМИ ДИСТАНЦІЙНОГО НАВЧАННЯ ТА КОНТРОЛЮ ЗНАНЬ / П.І. ФЕДОРУК // Математичні машини і системи, – 2006. – № 4.
16. Таран Т.А., Сирота С.В. Технология обучения понятиям в интеллектуальных обучающих системах // Новости искусственного интеллекта. – 2006. – №6.
17. Федяев О.И., к.т.н., доц, Жабская Т.Е., аспирант, Лямин Р.В. Система индивидуального обучения студентов агентно-ориентированного типа // Конференция – г.Киев, – 2008.
18. Лямин Р.В. Многоагентная система обучения студентов на кафедральном уровне / Р.В. Лямин // автореферат к магистерской работе [Электронный ресурс]. – Режим доступа: http://masters.donntu.ru /2008/fvti/lyamin/diss/index.htm
19. Вороной А.С., Егошина А.А. Средства интеграции онтологий предметных областей для баз знаний интеллектуальных обучающих систем // Искусственный интеллект – 2’2010 – C. 65-73