Topical issue
    The relevance of forecasting the balance of natural gas in the sections of time on the basis of supply and demand is certain, because reasonable estimates are an effective tool for long-term plan-schika and management energootrasli. The relevance of research appears in the application of the results for the operational and tactical swissagency. Research in this area are many experts of the gas industry, namely:
• Barkhudaryan I.G Oganesyan L.A - The application of mathematical methods of forecasting, modeling of natural gas;
• Kovalenko M.V, Mahkota K.V News - the use of neural networks in predicting the use of energy;
• Ronald H. Brown, Brian M. Marx and George F. Corliss, and S. Gill, Dzh.Deferrari
Upward
Methodology and research methods
    Of the existing methods of forecasting in the consumption of energy most commonly used statistical methods and heuristic methods of forecasting.
One of the common ways of constructing probabilistic forecasts is the regression relationships. Are being built, mostly one-parameter model, where the variable is the time factor. Such models are often called dynamic, they are more typical for the prediction, because there is solved the question of changing the main character - the time in the future.
To identify some patterns change over time, not only to determine the trend. This is necessary to study the internal structure of the deviations of the dynamic range of the trend found by using the correlation method.
A distinctive feature of time series is a correlation between adjacent rows of members. However, the methods of correlation analysis based on the assumption of independence of the elements of the statistical series. Therefore, to establish a close connection between levels of a number of speakers is determined by the autocorrelation coefficient.
most often occurs in the time series polynomial, exponential and harmonic component of the trend. The most suitable for the separation of the trend is the moving average method.
1. Use two modifications of the method of moving averages: simple smoothing is based on the formulation of a new series of simple arithmetic mean, calculated for certain periods of time;
2. Weighted smoothing consists in determining the weighted average number of points equal to the dynamics.
    When building a long-term forecasts using more modern technology forecasting, for example: neural networks, genetic algorithms.
In the study the problem of priority is to determine the period for which is forecast. In this case there is the following grading period:
        1. Short-term - the period of pre-emption from a few hours to months;
        2. Medium - from several months to a year;
        3. Long-term - from one year to 5 years or more.
    One of the most difficult from the methodological standpoint is the problem of long-term forecasting. Its complexity is due to objective difficulties into account qualitative changes of the object projection, reliable and systematic information which in retrospective period is often missing.
    Process of solving the problem of forecasting can be considered as a transformation of the initial information obtained through the collection of statistical data or analysis of the solutions of related problems. Statistical data describing the process of gas consumption, form a discrete dynamic range, obtained by recording data over a certain period (hour, day, week, month, year).
    Short-term outlook is necessary in order to supervisory control, where the prediction of hourly and daily gas demand is necessary for a rational redistribution of gas flows between customers within a day of the month. Medium-and long-term forecasts are planned developments with a view to identifying common trends in gas supply system under consideration economic region. The most appropriate application in order to predict the following methods:
        * extrapolation of trends;
        * modeling;
        * ratings.
    Studies have shown that daily and annual gas consumption modes are formed: weather (current and previous outdoor temperature), astronomical (day and night, weekdays) by regime (degree of loading and the structure of consumers), public (social structure of the population) and other factors. The need for the heuristic analysis is also conditioned by the presence of background information factors are not quantifiable and related to the classification of the type of information to view.
Upward
At the global level:
    Planning and forecasting are widely covered in the works of modern foreign
Authors Ronald H. Brown, Brian M. Marx and George F. Corliss, S. Gill, Dzh.Deferrari, R. Ackoff, I. Ansoff, H. Benveniste, L. Vogel, J. Bigelya, G. Veilleux, C. Gannt, P. Drucker, W. Doering , M. Porter,
F. Taylor, A. Faiola, R. Fulmer, G. Ford, and others. Or rather:
                1. Ronald H. brown, brian M. Marx, and George F. Corliss "Mathematical Models for Gas Forecasting"
                2. S.Gil, J.Deferrari. "Generalized Model of prediction of Natural Gas Consumption"
Upward
Description of the developed subsystems
    To construct the model were used for gas provided Khartsyzsk controlled gas supply and gasification. These are the values of the monthly gas consumption for the city between 1998 to 2009. That is over 12 years, which will continue to implement long-term prognosis.
Problem Statement:
        We are given a discrete samples {y (t1), y (t2 )..., y (tn)} in a sequence of times t1, t2 ,..., tn. The problem of forecasting is to predict the value y (tn 1) at some future point in time tn 1.
        To solve this problem we use the mathematical tools of artificial neural networks.
To do this:
1. to form the training set;
2. choose the configuration of the neural network;
3. teach the neural network;
4. test the neural network.
The general mechanism of the subsystem forecasting natural gas consumption shown in Figure 1
                                       
                   
                           
            Figure 1 - Mechanism of Subsystem forecasting natural gas consumption
(Animation: volume - 56.011 KB; size - 336h280; number of frames - 6, the delay between shots - 1000 ms delay between the last and first frames - 1000 ms, number of cycles of repetition - a continuous cycle repetition)
    Formation of the training sample.
    Evidence of gas consumption are ordered in time sequence, ie a time series. In order to determine the dependence between observations (separated by time intervals of one month), we calculated autocorrelation coefficient, which reflects, in essence, the usual correlation, calculated between the image of the time series of current and delayed values of the dependent variable. A correlation analysis revealed that a relationship exists between the projected value from the value in the previous time (month) and from the same month a year earlier. Thus, selected the following values of time series: y (tn-1) and y (tn-13) as input factors.
The same study showed the influence of the mean value of gas consumption over the forecast month and the previous one, this value is also included in the input data set.
The normalization of the data can result in disparate data to comparable ranges, as well as bring them closer to the uniform distribution, which increases the information content of the training examples. Of the possible options for the normalization was chosen following a linear normalization, which leads all the values to a common range [0,1] [3].
Thus, all values of the time series were normalized, and the input of the neural network serves only the values in the range [0,1]. [3].
    Selecting neural network architecture.
    To solve the problems of forecasting are best suited following types of neural networks: multilayer perceptron (MLPset) and radial-basis network (RBFset). [4]
But in most cases when solving the same problem size of the network will exceed the size of the RBF MLP. Network RbF incorporates a single hidden layer and network MLP in the general case - a few. However, [5], any function can be approximated by a three-layer network MLP c one hidden layer with any degree of accuracy. In this case the use of more levels is not appropriate, since at this time of training increases and forecast accuracy decreases. Thus, the problem of choosing the number of layers for both types of neural networks is not: as a network RBF, and network MLP must have one hidden layer.
Web RBF can only use a Gaussian activation function, or its modification. At the same time MLP has the ability to work with different functions, which gives more opportunities for experimentation in the process of building a neural network model.
In addition, the network RBF does not have the ability to extrapolate data by increasing the width of the range for the input data, as the network response attenuates rapidly with distance from the training set formed by the centers of classes.
The disadvantage of network MLP compared with RBF is the higher complexity of training due to the need for joint training of several layers of neurons.
Thus, as the core network was chosen MLP-network. As a tool environment by using an Mathlab 7.0 (neural network toolbox). The experiments, which resulted in the following selected neural network architecture, consisting of input layer, hidden layer, consisting of 7 neurons and output layer (Fig. 2). The following activation function: the hidden layer - hyperbolic tangent, at the output - linear.
                                           
                                                           
Figure 2 - Neural network architecture
Upward
How It Works and planned results
    Provided for 12 years, information has been used as follows: the data of gas consumption over 11 years are included in the training set, and the last (12) year - in the verification. After training the neural network performed its testing. Test results are presented in Fig. 3. In the first half of Fig. 3 shows the results of testing on the training set, and the second - the check. The rms error on the verification sample is 6.1579e-004. It should be noted that the forecast for the first three months performed significantly better than a year.
                        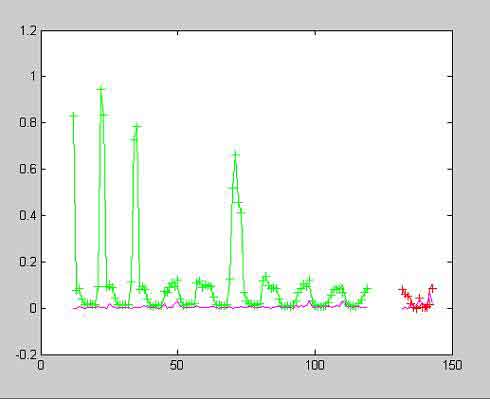
                                                           
Figure 3 - Testing the neural network.
Upward