
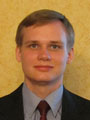

Segmentation and Recognition of Handwritten Dates
Author: M.Morita, R. Sabourin, F. Bortolozzi, C. Y. Suen
Source: http://www.cr-online.ru/data/articles/Segmentation%20and%20Recognition%20of%20Handwritten%20Dates.zip
Abstract
      This paper presents an HMM-MLP hybrid system to recognize complex date images written on Brazilian bank cheques. The system first segments implicitly a date image into sub-fields through the recognition process based on an HMM-based approach. Afterwards, the three obligatory date sub-fields are processed by the system (day, month and year). A neural approach has been adopted to work with strings of digits and a Markovian strategy to recognize and verify words. We also introduce the concept of meta-classes of digits, which is used to reduce the lexicon size of the day and year and improve the precision of their segmentation and recognition. Experiments show interesting results on date recognition.
1 Introduction
     Automatic handwriting recognition has been a topic of intensive research during the last decade. The literature contains many studies on the recognition of characters, words or strings of digits. Only recently the recognition of a sentence composed of a sequence of words or different data types has been investigated. Some applications on sentence recognition are reading texts from pages [1], street names from postal address [4] and date processing on cheques [5]. In such applications, usually a sentence is segmented into its constituent parts. In the literature two main different approaches of segmentation can be observed. The former and perhaps the most frequently used method segments a sentence into parts usually based on an analysis of the geometric relationship of adjacent components in an image while the latter uses an implicit segmentation which is obtained through the recognition process.
     In this paper we present an HMM-MLP hybrid system to recognize dates written on Brazilian bank cheques that makes use of an implicit segmentation-based strategy. In this application, the date from left to right can consist of the following sub-fields: city name, separator1 (Sep1), day, separator2 (Sep2), month, separator3 (Sep3) and year. Figure 1 details the lexicon of each date sub-field and Figure 2 shows some samples of handwritten dates. In such cases, the grey color represents the obligatory date sub-fields.
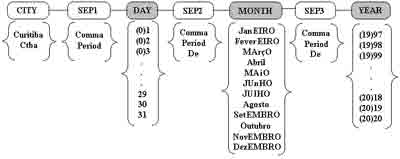
Figure 1. Lexicon of each date sub-field
      The development of an effective date processing system is very challenging. The system must consider different data types such as digits and words written in different styles (uppercase, lowercase and mixed). Although the lexicon size of month words is limited, there are some classes such as “Janeiro” and “Fevereiro” that contain a common sub-string (“eiro”) and can affect the performance of the recognizer. The system must also take into account the variations present in the date field such as 1- or 2-digit day, 2- or 4-digit year, the presence or absence of the city name and separators. Moreover, it must deal with difficult cases of segmentation since there are handwritten dates where the spaces between sub-fields (inter-sub-field) and within a subfield (intra-sub-field) are similar as shown in Figures 2(b) and 2(c). For example, in Figure 2(b) the intra-sub-field space between “1” and “0” is almost the same as the intersub- field spaces between “Curitiba” and “3” or “Fevereiro” and “10”. Therefore, it will be very difficult to detect the correct inter-sub-field spaces in this image using a segmentation based on rules.
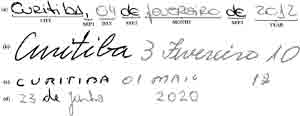
Figure 2. Samples of handwritten date images
      Hence, our system makes use of the Hidden Markov Models (HMMs) to identify and segment implicitly the date sub-fields. The three obligatory date sub-fields are recognized by the system (day, month and year). We propose to use Multi-Layer Perceptron (MLP) neural networks to deal with strings of digits (day and year) and HMMs to recognize and verify words (month). This is justified by the fact that MLPs have been widely used for digit recognition and the literature shows better results using this kind of classifier and HMMs have been successfully applied to handwritten word recognition.
      The main contribution of this work focuses on the strategy developed to segment the date sub-fields. It makes use of the concept of meta-classes of digits in order to reduce the lexicon size of the day and year and produce a more precise segmentation. Another important aspect of the system is the scheme adopted to reduce the lexicon size on digit string recognition to improve the recognition results. Such a strategy uses the information on the number of digits present in a string which was obtained through the HMMs as well as the meta-classes of digits. Besides, this paper presents the concept of levels of verification, and we show the importance of the word verifier in the system. Experiments show encouraging results on date recognition.
2 Definitions
2.1 Meta-Classes of Digits
      We have defined 4 meta-classes of digits (C0;1;2;3, C1;2, C0;9 and C0;1;2;9) based on the classes of digits present in each position of 1- or 2-digit day and 2- or 4-digit year (Figure 3). This is possible because the lexicon of the day and year is known and limited. While the class of digits C0-9 deals with the 10 numerical classes, the meta-classes of digits work with specific classes of digits. The objective is to build HMMs based on these meta-classes in order to reduce the lexicon size of the day and year and improve the precision of their segmentation. Besides, it can be applied to digit string recognition to increase the recognition results since very often confusions between some classes of digits can be avoided (e.g., 4 and 9, 8 and 0). The use of this concept on digit string recognition improved the recognition rate from 97.1% to 99.2% using a subset of hsf 7 series of the NIST SD19 database, which contains 986 images of 2- digit strings related to the lexicon of 2-digit day.
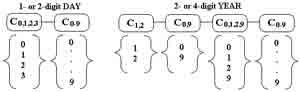
Figure 3. Classes of digits present in each position of 1- or 2-digit day and 2- or 4-digit year
2.2 Levels of Verification
      Takahashi and Griffin in [6] define three kinds of verification: absolute verification for each class (Is it a “0” ?), one-to-one verification between two categories (Is it a “4” or a “9” ?) and verification in clustered, visually similar categories (Is it a “0”,“6” or “8” ?). In addition to these definitions, Oliveira et al in [3] introduce the concepts of highlevel and low-level verifications. The idea of the high-level verification is to confirm or deny the hypotheses produced by the classifier by recognizing them. On the other hand, the low-level verification does not recognize a hypothesis, but rather determines whether a hypothesis generated by the classifier is valid or not.
      Based on these concepts, we propose to use an absolute high-level word verifier in order to improve the recognition results. The objective of the word verifier is to re-rank the N best hypotheses of month word recognition using a word classifier specialized in the specific problem: words instead of the whole sentence. The word recognizer takes both segmentation and recognition aspects, while the verifier considers just the recognition aspects. This verifier deals with the loss in terms of recognition performance brought by the word recognition module. In Section 3.3 presents more details about this verifier.
3 Description of the System
      In this Section we describe the modules of the system.
3.1 Segmentation into Sub-Fields
      A date image is first segmented into graphemes and then two feature sets are extracted. The segmentation algorithm and the features (global and concavity) are basically the same as that we have presented in [2]. However, here the features differ in the following aspects: both feature sets are combined with the space primitives, the sizes of the concavity feature vector and its codebook. Since the concavities have exhibited a good feature to improve the discrimination of letters and digits, we have used them in other parts of the system. They differ in the size of concavity vector and the zoning used.
      Both feature sets are combined through the HMMs that have been used to identify and segment implicitly the date sub-fields. The elementary HMMs used by the system are built at the city, space and character levels since each subfield with the exception of the city model is formed by the concatenation of space and character models. Considering that some sub-fields are optional and there is one model for each sub-field, we can have 8 possible date models which are formed by the concatenation of space and sub-field models.
      We have chosen an ergotic model with 5 states to represent globally the city names and noise (e.g., Sep1) and a linear topology to model spaces and characters such as letters and digits. The topology of the space models consists of 2 states linked by two transitions that encode a space or no space. We have considered 3 HMMs that model the intersub- field, intra-word and intra-digit spaces. The topologies of the character models consist of 4 or 5 states which were chosen based on the output of our segmentation algorithm. Considering uppercase and lowercase letters, we have 40 HMMs. For the digit case, we have defined 5 HMMs. The M0-9 model considers the 10 numerical classes and the other ones are defined based on the meta-classes of digits (e.g., the M1;2 model corresponds to the meta-class of digits C1;2 and so forth). The elementary HMMs are trained using the Baum-Welch algorithm with the Cross-Validation procedure [7]. Our training mechanism has two steps. In the first step, we train only the city model using 980 images of isolated city names. In the second one, besides the date database we have considered the legal amount database, which is composed of isolated words, in order to increase the training set. In this case, the parameters of the city model are initialized based on the parameters obtained in the previous step. Then, the other models present in the date and word images are trained systematically. We have used about 1,200 and 8,300 images of dates and words respectively.
      The month model consists of an initial state, a final state and 12 models in parallel that represent the 12 word classes. Each word model has two letter models (uppercase and lowercase) in parallel and 4 intra-word space models linked by 4 transitions. The same philosophy is applied to build the “de” separator model (Sep2 and Sep3). The day model consists of an initial state, a final state and the 2-digit day model in parallel with the 1-digit day model (Figure 5(a)). The 2- digit day model is formed by the concatenation of the models: M0;1;2;3, intra-digit space and M0-9. The 1-digit day model is related to the M0-9 model. The probabilities of being 1- (1D) or 2-digit (2D) day are estimated in the training set. The year model is built in the same manner.
      The segmentation of a date image into sub-fields is obtained by backtracking the best path produced by the Viterbi algorithm [7]. In this case, the system takes into consideration the result of the segmentation of the best date model (among the 8 possibilities) that better represents a date image.
3.2 Word Recognition
      The word probabilities are computed through the Forward procedure [7] for the 12 word models that we have used in the segmentation into sub-field module.
3.3 Word Verification
      A word image is first segmented into graphemes and then the following features are extracted: global, a mixture of concavity and contour and information about the segmentation points. The segmentation algorithm and the global features are the same as that we have employed in the segmentation into sub-field module. Since we are dividing a grapheme into two zones, we have two concavity vectors of 9 components each. For each vector, we have introduced 8 more components related to the information about the contour image to increase the discrimination between some pairs of letters (e.g., “L” and “N”). Thus, the final feature vector has (2 x (9 + 8)) 34 components. The segmentation features have been used to reduce confusions such as “n” and “l” since they try to reflect the way that the graphemes are linked together. Therefore, the output of the feature extraction is a pair of symbolic descriptions, each consisting of an alternating sequence of grapheme shapes and associated segmentation point symbols.
      Both feature sets are combined through the HMMs that have been used to verify the two best hypotheses generated by the word recognizer. We have adopted a similar architecture of the word models used in the word recognition, but here we are not modeling the spaces. The character models used to build the word models are based on the topologies of the character models described before, but in this case we are modeling the nature of the segmentation point (e.g., the transitions t12, t34, t57 and t67 of Figure 5(b)). The character models have been trained through the Baum-Welch algorithm with the Cross-Validation procedure using 9,500word images extracted from the date and legal amount databases.
      Figure 4 shows an example of how the word verifier interacts with the word recognizer. The word recognizer generates the list of hypotheses and the word verifier re-ranks the correct hypothesis (Novembro) to the top of the list by multiplying the probabilities produced by the word recognizer and verifier. The probabilities are computed by the Forward procedure. In Section 4 we will see the importance of the word verifier in the system.
3.4 Digit String Recognition (DSR)
      The number of digits supplied by the HMMs is used as information a priori on DSR to determine which of the 5 MLPs we have defined will be employed (Figure 6). The e0-9 classifier copes with the 10 numerical classes and the other ones are specialized in the lexicon of the meta-classes of digits (e.g., the e1;2 classifier works with the meta-class C1;2 and so on). This strategy aims at reducing the lexicon size on DSR to improve the recognition rates.
      The segmentation module is based on the relationship of two complementary sets of structural features, namely, contour & profile and skeletal points. The segmentation hypotheses are generated through a segmentation graph, which is decomposed into linear sub-graphs and represents the segmentation hypotheses. For each segmentation hypothesis a mixture of concavity & contour is extracted. Since we are dealing with multi-hypotheses of segmentation and recognition the generation of K best hypotheses of a string of digits is carried out by means of a Modified Viterbi, which ensures the calculation of the k best paths of segmentation-recognition graph. More details can be found in [3]. Thus, the final probability for a hypothesis of segmentation-recognition is given through the product of the probabilities produced by the classifiers (see Figure 4). For simplicity, this Figure presents just one hypothesis of segmentation. Afterwards, each hypothesis is submitted to the post-processor module, which verifies whether it belongs to the lexicon of the day or year.
3.5 Final Decision
      Since the date field is composed of three obligatory subfields, a date image is counted as correctly classified if these sub-fields are correctly classified.
4 Experiments and Analysis
      The system was capable to identify 95.5% on the test set, which is composed of 400 images, the best date model (among the 8 possibilities) that better represents a date image. Table 2 details the segmentation rate of each date subfield and the results when the number of digits is well estimated by the HMMs. The results shown in this Table were evaluated automatically by the system.
      Figure 7(a) shows an example where the date sub-fields are missegmented and Figure 7(b) demonstrates a difficult case of segmentation, where the spaces between sub-fields and within sub-fields are very similar. However, our approach succeeded in segmenting the date sub-fields correctly.
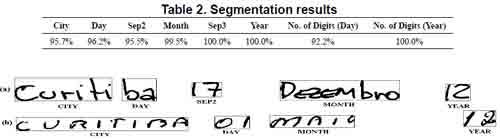
Figure 7. Examples of (a) missegmented and (b) well-segmented date images
5 Conclusion
      We presented in this paper an HMM-MLP hybrid system to recognize handwritten dates written on Brazilian bank cheques. The system makes use of the HMMs to segment the date sub-fields and considers different classifiers to recognize the three obligatory sub-fields. We also have introduced the concept of meta-classes of digits to reduce the lexicon size of the day and year and improve the precision of their segmentation and recognition. We have shown difficult cases of segmentation in which our HMM-based approach works well and interesting results on date recognition.
References
[1] U. Marti and H. Bunke. Using a statistical language model
to improve the performance of an hmm-based cursive handwriting
recognition system. International Journal of Pattern
Recognition and Artifical Intelligence, 15(1):65–90, February
2001.[2] M. Morita, A. E. Yacoubi, R. Sabourin, F. Bortolozzi, and C. Y. Suen. Handwritten month word recognition on Brazilian bank cheques. In Proc. 6th ICDAR, pages 972–976, Seattle- USA, September 2001.
[3] L. S. Oliveira, R. Sabourin, F. Bortolozzi, and C. Y. Suen. A modular system to recognize numerical amounts on Brazilian bank cheques. In Proc. 6th ICDAR, pages 389–394, Seattle- USA, September 2001.
[4] J. Park and V. Govindaraju. Use of adaptive segmentation in handwritten phrase recognition. Pattern Recognition, 35:245– 252, 2002.
[5] C. Y. Suen, Q. Xu, and L. Lam. Automatic recognition of handwritten data on cheques - fact or fiction ? Pattern Recognition Letters, 20(13):1287–1295, November 1999.
[6] H. Takahashi and T.D.Griffin. Recognition enhancement by linear tournament verification. In Proc. 2nd ICDAR, pages 585–588, Japan, 1993.
[7] A. E. Yacoubi, R. Sabourin, M. Gilloux, and C. Y. Suen. Off-line handwritten word recognition using hidden markov models. In L. Jain and B. Lazzerini, editors, Knowledge Techniques in Character Recognition. CRC Press LLC, April 1999.