Abstract
Содержание
- Introduction
- 1. Theme urgency
- 2. Statement of the problem
- 3. The purpose of the study
- 4. Overview of Research and Development
- 4.1 Overview of international sources
- 4.2 Review of national sources
- 5. Analysis of methods of predicting solar flares
- 6. The main stages of predicting solar flares
- 7. The processing algorithm MDI magnetograms of the Sun
- 8. The network cascade correlation
- Conclusions
- References
Introduction
From the point of view of astronomers, our sun - the star of calm. However, from time to time on it there are flashes, and the flow of hard electromagnetic radiation from the sun increases thousands of times. Conventional solar flares last only a few minutes. But it is enough to have serious consequences for life on Zemle.Uzhe eight minutes after the start of the outbreak invisible ultraviolet, X-rays and gamma rays reach the Earth's orbit. Streams of charged particles accelerated to energies of giant, and huge plasma emission suddenly descend into interplanetary space. Fortunately, the Earth's atmosphere protects us from harmful radiation, and its magnetic field - from charged particles. However, even in the world, and especially in space, solar flares are dangerous, they can cause serious problems for the aerospace industry, communication on the basis of electromagnetic and energy systems, radio broadcasts.
1. Theme urgency
Predicting solar flares more than a day before they occur, will provide an early warning to protect satellites, power grids and astronauts from potentially dangerous radiation. Satellites can for example be so designed that would be temporarily closed. Mains can also be reserved before the outbreak.
Until now, solar weather forecasts were made by hand: the experts looked at the two-dimensional satellite images of the sun and the eye was assessed on the probability of future aktivnosti.Poetomu there is a need for an automated system of predicting solar flares, which would allow the accurate forecasts based on recent data on the Sun's surface. Master's thesis is devoted to the actual scientific problem of developing an automated system for forecasting of solar flares.
2. Statement of the problem
The aim of the study is to develop an automated system that allows you to advance to obtain reliable predictions of solar flares in order to prevent serious problems in the aerospace industry, applied solar flares, as well as for the smooth operation of an electromagnetic communication and power systems, radio broadcasts.
This goal is achieved by solving the following problems:
- analysis of key information components, changes in the physical processes in the different structures of the solar atmosphere in the stages preceding the powerful solar events to maximize the predictive accuracy in the problem of short-term forecasting of solar activity;
- study of existing methods of classification of sunspots in order to achieve a high percentage of accuracy of forecasts
- Development of algorithm processing image sequences MDI magnetograms.
- dock design automation system

Where a - amplitude of the cycle associated with an increase in the minimum cycle time, s - asymmetry cycle, b - time in months from minimum to maximum
as the objective function is proposed to use the minimum difference in sunspot numbers.
| F (t) - F (t ') | -> min
where F (t) - the equation for estimating the number of sunspots for the data received from the base.
F (t ') - an equation for estimating the number of sunspots for the data received by the system
choice of such an objective function ensure compliance maximize predictive accuracy in the problem of short-term forecasting of solar activity. Constraints of the problem can be written as follows:
- Number magnetograms Ai must be> 0 at the time of the forecast;
Sum of Ai> 0, for each time period (i) - The period during which an estimate of the number of spots must be> 5 years.
T-T0> 5, at every moment of forecasting
where T0 - the start time of the flare activity,
T - time of the flare activity. - amplitude of the cycle and tends to min;
a-> min - Flash Output Bi must meet the cash register X or M
Amount of Bi> = 1,0 * 0,00001 W \ m2
3. The purpose of the study
aim of this study is to develop an automated system that allows you to receive advance forecasts of outbreaks in the sun in order to prevent problems in the aerospace industry, applied solar flares, as well as for the smooth operation of an electromagnetic communications and power systems, radio broadcasts.
subject of study span>: methods of predicting solar flares.
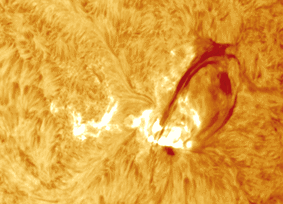
Figure 4.1 - Solar Flare M-Class
(animation: size - 133 KB size - 600x368, number of frames - 30, the delay between shots - 30 ms delay between the last and first frames - 100 ms, the number of cycles of repetition - 7)
4. Overview of Research and Development
Since the development of such a system is an important task it has occupied many spetsiality, both American and domestic. On the subject was protected by a lot of papers and theses, conducted a number of studies.
4.1 Overview of international sources
To date, the development of such a system are engaged in the British scientist R. Qahwaji Roger. Green. They have written a number of articles on this temu.Oznakomitsya they can be on their website; [2 - 6]. In their articles, they describe in detail the methods that were used in their studies and have reached at this time results.
Another way of predicting in their articles considered American scientists from Purdue University. The new warning system analyzes the differences in gamma radiation, which is emitted at the time of the collapse of the atoms of radioactive elements. This decay rate is constant, but the latest research results put this into question. Scientists have hypothesized that says that the rate of radioactive decay is changing under the influence of solar activity, possibly due to the flow of sub-atomic particles of neutrinos from the Sun. [6].
In his work H. Alomari. Mohammad and Stanley S. Ipson [7] described the introduction of the new study, The computerized fully, machine learning-based decision rules and models that can be used in the design of systems for automated space weather forecasting. Dock design of the system in this book consists of three steps: (1) Design Tools computer to find associations of solar events and features (2) the application of machine learning algorithms to data sets, associations, and (3) the study of the evolution of models of sunspot groups using time-series methods
4.2 Overview of national sources
In the Research Institute of Radio Physics Nizhny Novgorod, N.S. Bellyustin, A.A. Indeed, Y. Tikhomirov, O. Shemagin, A.N. Yashin propose a new method for predicting the powerful energy in the solar atmosphere on the solar disk image sequence preceding the powerful solar flare. The method uses the image feature extraction, most effectively separating previous outbreaks of images from other images, forecasting is provided by combining the selected features in the committees [9].
At Odessa National Technical University, S.G. Antoshhuk, O. Babilunga, AA Nicolenko consider the edge images of objects napolutonovyh image morphological processing method based on the hyperbolic wavelet transform. [10]
V.P. Maksimov in the works engaged in the research of space weather, solar vydklil determinants of space weather forecasting and objectives. [11].
5. Analysis of methods for predicting solar flares
Currently, there are a number of prediction methods, the most common methods are shown in Figure 1:

The most common methods of predicting solar flares are:
Prediction method using three-dimensional images obtained Solar Heliospheric Observatory
. This method involves the extraction of features that will be used to create the corresponding 3D-models. These models will provide a physical and visual descriptions of the features of interest, which would be more comprehensive than the current text descriptions and specifications of models. Prediction by this method does not allow to reach a high percentage of accuracy of prediction and requires considerably more time for the prognosis in comparison with other methods.
Prediction method by measuring the difference in the atoms of radioactive elements decay gamma rays. This prediction method is based on the hypothesis that the force decay depends on the solar activity, such as a flow of subatomic particles called neutrinos moving from the Sun. This effect may vary according to seasonal changes in the distance from the Earth to the Sun, as well as during solar flares. The disadvantage of this method is not very high percentage of prediction accuracy and is not possible to determine the class flare, which is an important factor in a lot of predicting solar flares.
Developed system of forecasting solar flares will be based on the method of prediction using neural networks, namely networks Cascade Correlation These method yields the most accurate predictions about the possible outbreak of the sun. Forecast accuracy using neural networks is around 80%.
6. The main stages of of forecasting of solar of outbreaks.
Milestones prediction using neural networks, is to ensure that, at the first stage, the treatment of MDI images of the sun for the detection of spots. The second stage - the classification obtained spots in the group. The third stage involves the application of neural networks to calculate the probability of an outbreak and identify its possible grade. The most best suited for this network Cascade Correlation, as it provides a better connection between solar flares and spots classes. Cascade Correlation Network - special multi-layer network (cascade) architecture, which allows her to teach in a constructive way: at the termination of the convergence of the network adds a new neuron and further modified by learning context, only the neuron. This approach allows us to determine the size of the network, adequate to the task at hand, and to reduce the computational cost of training.
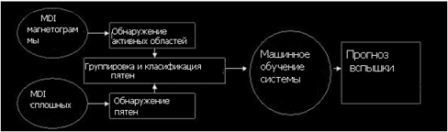
7. The processing algorithm magnitogrammnogo images of the Sun
MDI magnetograms (MDI-Michelson Doppler Imager) is a high-contrast black-and-white digital images, which encodes a photometric measure of the radial component of the magnetic field of the Sun with a spatial resolution of 2n (~ 1700) km \ pixel and time increments 96m. The white areas on the magnetogram corresponds to the strong negative field, black-strong positive, the gray area is characterized by a magnetic field close to 0.
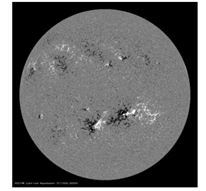
MDImagnitogramma of the Sun
Idea MDI treatment is to implement the following steps:
- Segmentatsiya.Fragment of the magnetogram, containing the JSCs, is represented as a a digital image of, each pixel x of which, is encoded by tensions the radial components of the magnetic of the field a level of "of gray» I (x). Thus, magnetogramm seems like a digital brightness function I (x): RxZxZ-> R, defined on the lattice of the x belongs to the domain ZxZ. Pixels above the level of "gray» I (x)> = h are marked with black and belong to the class of "strong positive area" pixels is less than the level of "gray» I (x)> = h are marked with white and belong to the class of "strong negative region . " The remaining pixels are marked by gray and are considered neutral.
- Search active region. At this stage, searched the area, which is a timber Most active based on the selected criteria. The criteria will be considered a rectangular area defining strongly negative and positive field.
8. The network cascade correlation
The network suits us due to the fact that the topology of these networks is not fixed. Cascade Correlation network - a multi-layered network that is trained in a constructive way, ie, to the extent of training the network adds new neurons.
This learning algorithm is designed so that trains in fact, only one layer of trainees links that allow you to limit the simplified (and more computationally simple) implementation of the back-propagation algorithm. The given a network of quickly is trained, with the majority learning parameters (such as architecture, size of the network or the parameters of training speed) are determined by by the very process of training. The algorithm begins with a simple initial approach, which is a network trained with one layer of ties.
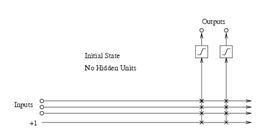
initial approximation is trained to minimize the mean square error:
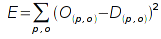
One of the major problems facing these networks is a more fitting training data, especially when dealing with real problems (Smieja, 1993). On the other hand, the Cascade-Correlation architecture has several advantages. Firstly, she learns very quickly, at least in 10 times faster than algorithm with error backpropagation (Shet et al., 2005). Second, the network determines its own size and topology, and it retains the structure, even if it has created a training set of changes (Fahlmann and Lebiere, 1989). Third, it requires no back-propagation signal through a communication network (Fahlmann and Lebiere, 1989). Finally, this structure is useful for incrementally learning in which new information is added to the trained network (Shet et al. 2005).
Can be concluded that the network cascade correlation ensure optimal performance of neural networks to handle solar data directories.
Conclusions
The study of solar flares is necessary to develop a scientifically sound and reliable forecast of the radiation situation in the near space. In this practical problem in the theory of outbreaks. It is important, however, and more. Solar flares must be studied to understand the various flare phenomena in space plasma. Unlike flares on other stars, as well as many other similar (or seemingly similar) transient phenomena in the universe, solar flares most comprehensive study available in almost all electromagnetic spectrum - from the kilometer-long radio waves to hard gamma rays. Physics of solar flares - a kind of cut across many areas of modern physics: the kinetic theory of plasma to high-energy particle physics.
modern space observations allow us to see the emergence and development of a solar flare in the UV and X-rays with high spatial, temporal and spectral resolution. A huge stream of observational data on outbreaks and resulting effects on the atmosphere of the Sun, interplanetary space, the magnetosphere of the Earth's atmosphere and gives the opportunity to thoroughly check all the results of theoretical and laboratory modeling of outbreaks.
Further research is focused on the following aspects:
- Quality improvement scolded forecasting method, its complement and extension.
- Improved accuracy to make predictions.
In writing this essay master's work is not yet complete. Final completion: December 2013. Full text of the and materials on the topic can be obtained from the author or his manager after that date.
References
- O. Ahmed, R. Qahwaji, T. Colak, P. Higgins. P. Gallagher and S. Bloomfield,
Solar Flare Prediction using Advanced Feature Extraction, Machine Learning, and Feature Selection
, Solar Physics, Springer, 283 (1): 157-175, 2013. - Tsagour I and et al ,
Progress in space weather modeling in an operational environment
, Space Weather and Space Climate, 3, 2013. - O. Ahmed , R. Qahwaji,T. Colak, T. Dudok de Wit and S. Ipson,
A New Technique for the Calculation and 3D Visualisation of Magnetic Complexities on Solar Satellite Images
, Visual Computer, DOI: 10.1007/s00371-010-0418-1 , 26 (5): 385-395, 2010. - T. Colak and R. Qahwaji, "Automated Prediction of Solar Flares Using Neural Networks and Sunspots Associations", 11th Online World Conference on Soft Computing in Industrial Applications (WSC11), 2006.
- R. Qahwaji, "Machine-Based Automatic Detection and Verification of Solar Features", International Conference on Telecomputing and Information Technology (IEEE ICTIT), pp. 87 - 91, Amman, Sep 2004.
- RDNews.[Электронный ресурс]. – Режим доступа: http://rnd.cnews.ru/news/line/index_science.shtm .
- Аpplied Machine Learning for Solar Data Processing: Developing Automated Technologies for Knowledge Extraction and Prediction of Solar Activities using Machine Learning/ Mohammad H. Alomari,Stanley S. Ipson – LAP LAMBERT Academic Publishing, 2011. – 152 pages.
- Земля и вселенная.[Электронный ресурс]. – Режим доступа: http://ziv.telescopes.ru/rubric/astronomy/index.html .
- Московский государственный уиверситет.[Электронный ресурс]. – Режим доступа: https://docs.google.com/viewer?a=v&q=cache:VteCw-EN8OoJ:dlaptev.org/papers/Laptev11 .
- Институт прикладной физики РАН, Нижний Новгород.[Электронный ресурс]. – Режим доступа: http://webcache/library.mephi.ru/data/scientific-sessions/2011/neiroinform .
- Одесский технический университет.[Электронный ресурс]. – Режим доступа: http://archive.nbuv.gov.ua/portal/21.pdf.
- Космофизика.[Электронный ресурс]. – Режим доступа: http://www.kosmofizika.ru/irkutsk/maksimov.htm .
- А. Орлов, Архитектура и обучение сети Каскадной Корреляции.[Электронный ресурс]. – Режим доступа: http://neural.ru/dictionary/.