Abstract
- Introduction
- 1. Description of the subject area
- 2. The urgency of the problem
- 3. The theoretical part
- 4. Planned practical results
- Conclusion
- References
Introduction
In recent years, intensive development of hybrid intelligent systems to take advantage of traditional means and methods of artificial intelligence, and, at the same time, overcoming some of their shortcomings, can solve problems unsolvable by individual methods of artificial intelligence. Hybrid intelligent systems can more effectively combine formalized and formal; knowledge through the integration of traditional means of artificial intelligence.
Such a variety of intelligent systems is determined by the need to formalize a variety of data and knowledge, and not always for the formalization of the process can be successfully performed for data and knowledge of any kind. That is why in recent years began to develop intensively hybrid intelligent systems (a type of multi-component of intelligent systems) – take advantage of the traditional means of artificial intelligence, and at the same time overcoming some of their shortcomings, can solve problems unsolvable by individual methods of artificial intelligence. Hybrid intelligent systems enable more efficient and formal; connect formalized knowledge through the integration of traditional means of artificial intelligence.
Description of the subject area
Since that time, were created by different types of artificial intelligence systems such as expert systems, fuzzy systems, decision support systems, artificial neural networks, robot motion planning, genetic algorithms.
The use of hybrid intelligent systems particularly useful in medicine, banking, in pattern recognition, detection of faults in technical systems, etc., that is, in those sectors where we have to deal with both crisp and with fuzzy knowledge.
The urgency of the problem
If you argue from the standpoint of the theory of HIS systems, the primary in artificial intelligence, the principle of a systematic approach has been violated. None of the methods can not, and should not claim to be universal. Advantages some ideas compensate for weaknesses in other parts of the interaction of the whole, and give new, integrative property qualitatively new entity – HIS.
The above arguments are talking about the prospect of hybrid intelligent systems, both in terms of increasing the relevance of models of the originals, as well as reducing the time to create projects of information systems.
Object of research: methods of construction of hybrid intelligent systems.
Subject of research: practical implementation of the algorithm for constructing hybrid intelligent systems.
The theoretical part
In order to implement the method of constructing a hybrid intelligent system, you need to learn the basic concepts, analyze the existing methods and identify among them those that are more efficient and need to be further developed.
Planned practical results
On the basis of all the above model was developed hybrid intelligent multi-agent learning system, which is organized as a community of interacting groups of agents. The composition of the proposed subsystem competence assessment is a step in Figure 1.
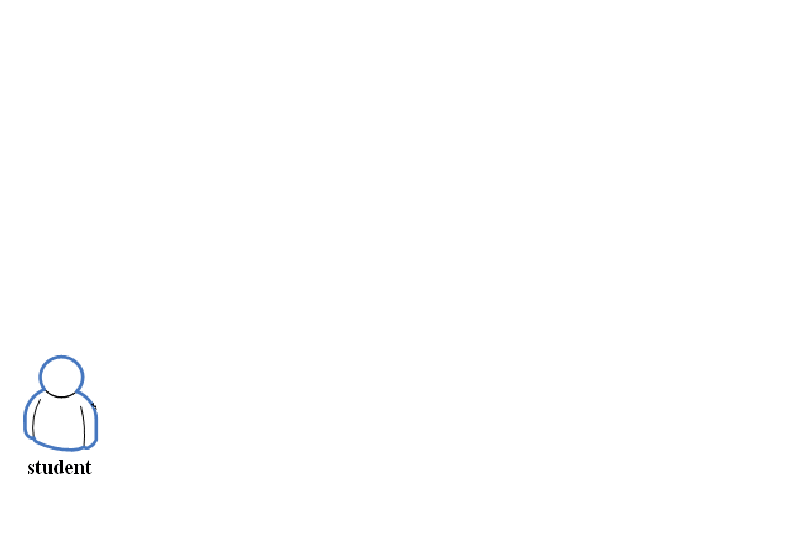
Figure 1 (animation - 26 shots, 10 reps) - show the functional diagram of hybrid opto-electronic system step by step
Subsystem competency assessment the following tasks. The first time the system is formed and the student profile is determined by its initial level of knowledge to be filled on the basis of their core competencies map to start learning. At this stage AMCC and DPS receives information from collective training of agents (CTA). After the completion of the next stage of learning or teaching selecting competency testing AMCC and DPS interacts with AMCS, using a competency model that establishes the missing competence to determine the level of knowledge on this competence. For this trajectory is formed by individual testing AFCC and AFITT, creates individual test environment AITE and formed a benchmarking exercise to test AFSA. To fill the test environment used test assets RRT.
Training can include working with basic training courses (for the formation of the required competencies), and with additional training courses formed - to address the identified gaps that do not allow the student to learn a new course. The solution of the re-formation of the individual course of study may involve several steps and performed CTA. In the initial step is a comparison of the desired (reference) with the competence of the current (original) The jurisdiction of the student. At discrepancy queries the repository to find the set of learning objects, learning is necessary for the formation of the required competencies. After the training phase conducts testing on selected competence. According to test results formed the required state for the next step of planning, etc. The condition for the completion of the planning will be to eliminate the differences between the current and desired levels for the last step planning competencies.
To better assess the knowledge to be used for the required competence ontology and on that basis to build customized learning paths and testing.
Conclusion
Hybrid intelligent systems - a new and promising area of fundamental interdisciplinary research focused on solving complex practical problems with the use of knowledge integration and synergies. The basic idea is to eliminate attempts to find and use a universal tool for solving the problem and the transition to its design as a combination of artificial intelligence, operations research, decision theory and systems analysis. In this case, the computer is not used as a means of implementing the known methods, but as a means of synthesis methods that are relevant to the dynamic composition and structure, heterogeneous subjective entities - a complex practical problems.
Analysis of the status of the theory, methodology, and technology of hybrid intelligent systems shows that there is still much to do. However, the first theoretical and methodological results discussed in this review article and other works of the author in the list of the available literature, helped solve some difficult problems of planning.
Summarized in the paper, the results strongly suggest that HIS fundamentally able to solve problems that can not be modeled within a single method, accelerating the creation of intelligent systems is several times lower forecast error, improve the quality of both tactical and strategic planning.
The results of modeling and HIS three subsystems integrator indicate the possibility of its creation in MatLab and the reliability of the solutions. The implementation of the integrator in the form of a Kohonen neural network, learning "without a teacher," preferably with a small number of snapshots.
Experimental realization of models with HIS implementation in two ways integrator (based on multi-layer neural network and Kohonen network) demonstrates the versatility and modular structure, the need for selecting the parameters of the system, depending on the size and nature of the input and output of data in a particular subject area.
HIS contains a synergistic combination of neural, fuzzy, and character, and the other models in order to achieve its full range of cognitive and computational capabilities.
Implementation of HIS in MatLab has a number of drawbacks: it is required expensive commercial software package MatLab, which uses only a small part of its functionality. A method for implementing HIS depends on the application, volume and nature of the input data, requires a highly skilled and long terms of its creation.
The proposed system can be used to enhance learning competency-based approach. The use of a repository of test resources will accumulate test items for the required competencies and based on them to build customized tests. Use of this system will automatically generate a sequence of educational material - the main program and re-training, according to the learner's current competence.
In order to reduce the cost and timing of the development of HIS for a particular domain is advisable to create an instrumental intellectual environment of its design (without using MatLab), providing a rapid prototyping based HIS principles of CASE-technology.
References
- Гаврилов А.В. Гибридные интеллектуальные системы / А.В. Гаврилов – Новосибирск: НГТУ, 2003. – 168 с.
- Попов Э.В. Экспертные системы / Э.В. Попов – М.: Наука, 1987. – 450 с.
- Венда В.Ф. Системы гибридного интеллекта / В.Ф. Венда – М.: Машиностроение, 1990. - 282 с.
- Нильсон Н. Принципы искусственного интеллекта / Н. Нильсон – М.: Радио и связь, 1985. – 91 с.
- Ярушкина Н.Г. Основы теории нечетких и гибридных систем / Н.Г. Ярушкина – М.: Финансы и статистика, 2004. – 320 с.
- Medsker L.R. Hybrid Intelligent Systems / L.R. Medsker – Boston: Kluwer Academic Publishers, 1995. – 298 p.
- Клачек П.М., Корягин С.И. Гибридные адаптивные интеллектуальные системы / П.М. Клачек, С.И. Корягин, А.В. Колесников, Е.С. Минкова – Калининград: БФУ им. И. Канта, 2011. – 374 с.
- Wermter S., Sun R. Hybrid Neural Systems / S. Wermter, R. Sun – Heidelberg, Germany: Springer Verlag, 2000. – 102 р.
- Колесников А.В. Гибридные интеллектуальные системы: Теория и технология разработки / А.В. Колесников – СПБ: Изд-во СПбГТУ, 2001. – 711 с.
- Simon Н. А. Information processing models of cognition / Н. А. Simon // J. Amer. Soc. Information Science. – Sept. 1981. – 208 p.
- Gavrilov A.V. An architecture of neurocomputer for image recognition / A.V. Gavrilov // Neural Network World. – 1991. – N 1. – p. 59–60.
- Hopfild J.J. Neural networks and physical systems with emergent collective computational abilites / J.J. Hopfild // Proc. Nat. Acad. Sci. USA. – 1982. – p. 2554–2558.
- Колесников А. В., Кириков И. А. Методология и технология решения сложных задач методами функциональных гибридных интеллектуальных систем / А. В. Колесников, И. А. Кириков – М.: ИПИ РАН, 2007. – 387 с.
- Смирнов А.В. Онтологии искусственного интеллекта: способы построения и организации / А.В. Смирнов, М.П. Пашкин, Н.Г. Шилов, Т.В. Левашова // Новости искусственного интеллекта. – 2002. – № 1. – С. 3–13.
Important
This master's work is not completed yet. Final completion: January 2014. The full text of the work and materials on the topic can be obtained from the author or his head after this date.