Essay on the topic of final work
Contents
- Introduction
- 1. Relevance of the topic
- 2. Purpose and objectives of the study, planned results
- 3. Definition of Generative Modeling
- 4. Generative modeling methods
- 5. Generative Modeling Tools
- 6. Benefits of Generative Modeling in Industrial Design
- Conclusions
- List of sources
Introduction
Industrial design, as a key component of successful product manufacturing, plays an unparalleled role in ensuring not only functionality, but also outstanding aesthetics and usability. It is an integral part of today's industry, where continuously evolving trends highlight the growing influence of generative methods and tools in creating truly innovative products.
Today's consumers are increasingly demanding of products not only in terms of functionality, but also design. Industrial design, in this context, becomes not just a means of shaping the appearance of goods, but a real tool that determines the competitiveness of products on the market.
Given these challenges, modern designers are increasingly turning to generative modeling, which opens up new perspectives for creativity and innovation. Generative modeling in industrial design not only automates processes, but also provides unique opportunities to optimize production cycles, reduce costs, and create unique shapes that bring a new level of originality to the world of products.
1. Relevance of the topic
Nowadays, modern industry is faced with an increasing need for highly efficient tools that can effectively adapt to dynamic market demands. In this context, generative modeling emerges as a promising direction that is actively integrated into industrial design processes.
This innovative methodology provides the opportunity to combine creativity with cutting-edge technology, creating a new level of opportunity to develop products that not only meet high standards of functionality, but also have outstanding design. As a result of this combination, innovative products emerge that can successfully compete in the market, attracting attention and satisfying the needs of the most demanding consumers.
Generative modeling, with its ability to automate and accelerate design processes, gives industrial enterprises a significant advantage in reducing development time and increasing overall production efficiency. This opens the door to rapid innovation and gives businesses the flexibility to respond to changing market demands.
2. Purpose and objectives of the study, planned results
The study aims to identify the potential and effectiveness of generative modeling in the field of industrial design to optimize production processes and create innovative products.
Main objectives of the study:
- Exploring the Fundamentals of Generative Modeling: Review existing generative modeling methods and tools in the context of industrial design.
- Analysis of the use of generative modeling in industry: Explore successful cases of using generative methods in various industries.
- Prototype development using generative modeling: Create a prototype of a design product using selected generative modeling methods and evaluate the results.
- Evaluating the effectiveness of generative modeling: Compare the design process and results using generative modeling and traditional methods.
Object of study: generative design methods
Subject of research: implementation of generative modeling in industrial design processes in order to optimize and improve the efficiency of production processes.
Planned results:
- Advanced understanding of generative modeling: Analysis of key methods and tools and their applicability to industrial design.
- Practical application: Development of a product prototype that confirms the capabilities of generative modeling in creating innovative formsm and structures.
- Assessing Benefits and Limitations: Gain insight into the benefits and limitations of generative modeling in industrial design to help inform decisions in future designs.
1. Definition of Generative Modeling
Generative modeling is an innovative approach that uses various algorithms and computer programs to automatically generate and optimize design concepts. This method is widely used in the field of industrial design, where its benefits go beyond a simple automated process.
The generative modeling process involves the use of algorithms that can generate a variety of design options given predefined parameters and constraints. These algorithms can work with various types of data, including geometric, structural, functional and other characteristics.
One of the key benefits of generative modeling is its ability to quickly generate and evaluate a large number of design options, allowing for an efficient iterative development process. This allows designers and engineers to quickly and efficiently explore different concepts, find optimal solutions, and reduce development time.
In addition, generative modeling facilitates the creation of innovative and unique design solutions, often going beyond traditional approaches. This allows organizations and design professionals to achieve outstanding results while maintaining competitiveness in the market.
Generative modeling can be successfully applied not only in industrial design, but also in various fields such as architecture, art, and even science. Its versatility and adaptability make this approach an essential tool for those seeking innovation and optimization in the creative and design processes.
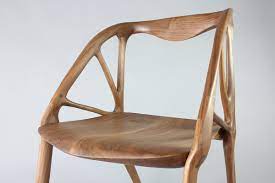
2. Generative modeling methods
- Parametric design is an innovative method based on the determination of key parameters that control not only the shape, but also the structure of the object. Artificial changes in these parameters automatically and instantly lead to corresponding changes in the design, which greatly simplifies the process of experimenting with different options. This technology also enables rapid response to dynamically changing requirements, a key benefit in today's development and design environment.
- Genetic programming, in turn, is an evolutionary approach to creating design solutions. In this method, program structures are developed and improved based on specified criteria. This process of software evolution allows the generation of complex and adaptive designs that can effectively solve a variety of problems. Genetic programming opens up opportunities for creating innovative solutions that adapt to changing environmental conditions.
- Evolutionary algorithms, inspired by natural selection, represent another approach to the evolution of design solutions. Using methods of combination, mutation and selection of the best characteristics, evolutionary algorithms boldly aim to create optimal designs. These algorithms are able to adapt to given conditions, striving for outstanding results in the context of the given tasks and requirements. This approach allows you to effectively solve complex design problems and provides a high degree of flexibility in finding optimal solutions.
3. Generative modeling tools
- Rhino Grasshopper is a preeminent tool in the field of parametric modeling, providing designers with the unique ability to create complex shapes and structures that are easily manipulated using parameters. This popular tool has become an integral part of the creative process, providing flexibility and efficiency in design.
- Autodesk Generative Design, in turn, stands out as an advanced platform that embodies the principles of artificial intelligence to create thousands of designs. This platform not only generates many possible concepts, but also automatically determines the optimal solutions, which significantly speeds up the design process and improves the quality of the final product.
- MATLAB, a powerful mathematical modeling software, provides extensive capabilities for implementing evolutionary algorithms and genetic programming. Using this instools, designers and engineers can perform complex mathematical analyses.
- Efficiency: Increasing the efficiency of processes involved is a critical aspect of modern technology, particularly in the context of accelerating design and decision-making processes. The use of advanced technologies allows not only to reduce design time frames, but also to significantly improve the overall efficiency of strategic decision-making.
- Innovation: One of the key factors contributing to innovative development is the ability to create unique and modern forms. Integrating modern technologies into design processes allows us to expand the boundaries of creativity and bring to life ideas previously considered unattainable. This promotes variety and originality of products, which in turn can significantly increase their competitiveness in the market.
- Optimizing production processes and product performance becomes more accessible through automated optimization. Technologies of data analysis, machine learning and artificial intelligence allow for detailed research and optimization in real time. This helps to increase production efficiency, reduce costs and improve the performance of the final product.
- Adaptability: Adaptability to changing requirements and conditions is an integral part of modern business. Technological solutions that provide a quick response to changes are becoming a key tool for the successful functioning of enterprises. The ability to quickly adapt to new market requirements and changes in external conditions allows you to remain competitive and successfully build business strategies.
4. Benefits of Generative Modeling in Industrial Design
Conclusions
The presented research is aimed at a deeper substantiation of the relevance and prospects of generative modeling in the context of industrial design. The main objective is to identify and analyze in detail the potential of this approach in order to determine its effective applications in industry. This research seeks to uncover the wide range of generative modeling capabilities, providing a comprehensive view of innovative technology solutions that can significantly improve production efficiency and product quality.
When writing this essay, the master's thesis has not yet been completed. Final completion: April 20224. Full text of the work and materials on the topic can be obtained from the author or his supervisor after the specified date.
List of sources
- Komarova A.A. Formation of architectural form using algorithmic methods / A.A. Komarova, S.V. Pykhtyuk, D.A. Chernyshov, M.E. Dymchenko // Engineering Bulletin of the Don, No. 8 (59), 2019. – P. 9.
- Salekh M.S. Introduction of digital methods at various stages of digital design / M.S. Saleh // Architecture and modern information technologies, No. 1 (54), 2021. – P. 268-278.
- Bzhakhov M.I. Algorithmic design in architecture / M.I. Bzhakhov, M.M. Efimova, A.V. Zhurtov // Engineering Bulletin of the Don, No. 2 (49), 2018. – P. 166.
- Potapenko A.A. Algorithmic design as a means of forming analytical and design models in architecture / A.A. Potapenko // Architecture and design: history, theory and innovation, No. 5, 2021. – P. 307-311.
- Hopcroft D. Introduction to the theory of automata, languages and computations / D. Hopcroft, R. Motwani, D. Ullman. – M.: Williams Publishing House, 2002. – 528 p.
- Bessarabova E.V. Three-dimensional modeling of industrial and architectural objects / E.V. Bessarabova // Modern science-intensive technologies, No. 4-2, 2016. – P. 225-229.
- Chen A., Tao Y., Kang R., Wang G., Grossman T., Coros S., Hudson S.E. “Forte: User-Driven Generative Design”, CHI 2018, April 21–26, 2018, Montreal, QC, Canada. – P. 496. Retrieved from https://www.researchgate.net/publication/324671677_Forte_User-Driven_Generative_Design.
- Li J, Kim J., Chen X. “Robiot: A Design Tool for Actuating Everyday Objects with Automatically Generated 3D Printable Mechanisms”, UIST '19, Session 6A: Fabrication, October 20–23, 2019, New Orleans, LA, USA. – P. 673-685. Retrieved from https://arxiv.org/pdf/2007.11199.pdf.
- Oh S., Jung Y., Kim S., Lee I., Kang N. “Deep Generative Design: Integration of Topology Optimization and Generative Models”, Journal of Mechanical Design, Vol. 141 (11), 2019, P. 111405-1-13. Retrieved from https://www.researchgate.net/publication/334472895_Deep_Generative_Design_Integration_of_Topology_Optimization_and_Generative_Models/link/60269ee0a6fdcc37a8217a39/download.
- Titova M.A. Generative design based on topology optimization using deep learning / M.A. Titova, A.Yu. Gromov // News of Tula State University. Technical Sciences, No. 2, 2022. – P. 246-248.
- Hattab M., Hamzeh F. “Analyzing Design Workflow: An Agent-based Modeling Approach”, Procedia Engineering, Vol. 164, 2016 – P. 510-517. Retrieved from https://www.researchgate.net/publication/311360797_Analyzing_Design_Workflow_An_Agent-based_Modeling_Approach
- Stieler, D., Schwinn, T., Leder, S., Maierhofer, M., Kannenberg, F., & Menges, A. (2022). Agent-based modeling and simulation in architecture. Automation in Construction, 141, 104426. Retrieved from https://doi.org/10.1016/j.autcon.2022.104426