FRACTAL
PROPERTIES OF MICROGEOMETRY
OF
PROCESSED SURFACES
Research manager: Ph.D. Dr.
Matviyenko Andriy

As
it is known creation of surfaces with the certain microgeometrical
properties is one of the primary goals of mechanical engineering.
Especially it concerns surfaces of particularly responsible products
of avia-space technics, instrument making, precision machine-tool
construction, etc. Moreover, the question of maintenance of
microgeometry of a surface is especially actual at development of
new technologies of processing of surfaces both mechanical, physical
and chemical ways of processing in the field of micro-and
nanotechnology.
Quality
of a surface is traditionally characterized by a roughness – an
arithmetic mean deviation, the maximal height of roughness, average
step of roughness of a structure, etc. and physicomechanical
properties of a superficial layer []. The roughness of a surface, as
many researchers have shown, is one of the basic parameters of
quality of a surface. In many cases the microgeometry of a
superficial layer predetermines behaviour of a surface during its
operation, and in case of micro- and nanotechnology the roughness is
considered not as secondary structure, but as a property of the
structure of material.
As
a rule, the microgeometry of a superficial layer is considered as a
certain static object which was generated during some influence.
Classically in processing of materials by cutting the roughness is a
geometrical prototype of a trajectory of moving of the tool which is
set by kinematics and modes of processing. On the other hand in the
processing power, temperature and other fields are formed, there is
a chemical influence on a surface, there are high pressures in a
zone of contact of the tool and a processable surface, movement of
dispositions, etc. owing to what the structure of a superficial
layer changes. Therefore formation of a surface on the whole and
microgeometry in particular is a result of action of set of
processes and not just merely geometrical "responses" of
action of the tool as for example it is marked in the work [2].
Within
the limits of the considered concept of "collective"
formation of a roughness it is possible to tell that such object as
the microgeometry of a surface is dynamic system. Studying of
dynamic system assumes studying of its properties which are defined
by some people invariants (for example, Lyapunov's parameter,
entropy, etc.). Therefore in this case the use of classical
geometrical parameters of a roughness is insufficient or simply
impossible. Moreover, geometrical parameters do not display such
important property of roughness as dynamic system – evolution.
Thus,
new approaches in an estimation of microgeometry of a surface are
necessary and one of such approaches can be a use of the theory of
fractals. Application of the theory of fractals will allow bringing
of a new parameter for an estimation of a roughness, to create base
not only for fractal classifications, but forecasting of change of
microgeometry during its formation, both at a stage of processing by
technological method, and at a stage of operation. The purpose of
the given work is check of the introduced assumptions from positions
of the theory of fractals on an example of formation of
microgeometry of a surface at a stage of technological influences.
Brief
positions of the theory of fractals
In
general fractal is a geometrical object (a line, a surface, a
spatial body) described by irregularity (structures, geometry,
etc.), but self-similarity (or symmetry). Self-similarity means that
the object more or less is uniformly arranged [3,4]on various scales
of its consideration. That is the invariance of the basic
geometrical features of object is supposed at the change of the
scale. For
example, in figures formation(education) фрактальных
objects is shown by a method of iterations.
http://www.cs.wisc.edu/~ergreen/honors_thesis/examples.html
Naturally
there are borders of these scales connected with concept of final
"weight" of object. The irregularity of object, in
general, means its some fractional dimension distinguishing it from
dimension of a line, a surface or space. Therefore in the theory of
fractals concepts of topological dimension are used so-called
Hausdorf-Bezikovich’s dimension which characterize
"deviations" of a fractal (object) from ideal topology.
Thus, fractals can be considered as set of the points enclosed in
space.
Fractal dimension is one of the basic characteristics
of a fractal. The central place in definition of fractal dimension
D (Hausdorf-Bezikovich’s
dimension) borrows concept of distance between points in
space. Hence, for definition D it is necessary to
measure "size" of set of points in space. The simple way
to measure length of curves, the area of surfaces or volume of a
body is to divide a body into small pieces, rectangulars, cubes or
spheres. Counting up number of these
elements which are necessary for a covering of required set
of points, we receive a measure of size of set.
Fractal geometry is based on
the experimental fact, that generally the length L of any
curve (which can be broken in any point) in the sedate image depends
on scale of measurement:
L
= C⋅δ1-D
where C is
a dimensional multiplier for each curve, D - fractal
dimension.
Other
characteristic of fractals is correlated dimension. Correlated
dimension D2 is defined by a ratio [26,
28]:
,
where
pi2
is
probability of at random taken point which belongs to i-th
cell (cube).
- the minimal number of n-dimensional cubes with an edge e, which are necessary for a covering of geometrical
object.
Correlation dimension can be presented in the form of
[4]:
,
where
I (e)
– the pair correlation integral defined from expression:
,
where
Q()
– Heviside’s function;
rn, rm
– radiuses-vectors of pair of points n and m respectively.
The
paired correlated integral defines probability of that two at random
taken points are divided by distance which is smaller than e. Besides

i.e.
fractal dimension D2 defines
dependence of paired correlated integral from e.
Thus,
dimension D2 is defined by the value of
correlated integral describing relative number of pairs of points
(n, m), removed on distances
smaller e.
The
following characteristic of fractal
properties of object is
correlated entropy or information
dimension which to some extent considers frequency of hit of a point
of set in an element of splitting of object.
Numerical value entropy
is the
quantitative characteristic of a degree of a chaotic state of
system.
Correlates
entropy
D1
is defined from the following expression [4]:
,
where
- entropy
of fractal sets,
- probability of a
finding of a point of object in i-th cell of splitting of object.
Correlated entropy can be calculated through correlated integral.
As
a rule, a definition of correlated dimension and entropy
is carried
out for multifractals – non-uniformed fractal
objects
which have not only geometrical, but also statistical
characteristics. In other words non-uniformed fractals have
non-uniformed distribution of points of set or different density of
"population" of set. Therefore during the research of
multifractals they speak about generalized fractal dimensions [3,4]
which can be presented above mentioned
fractal
dimensions. But in connection with special specificity of
multifractals for the analysis the function of multifractal
spectrum
or a spectrum of a multifractal singularity
is used.
As it is mentioned in the work [4],
the size of function a multifractal
spectrum is actually equal
to Hausdorff’s dimension
(D) of some homogeneous fractal subsets from
initial set which gives the dominating contribution to statistical
characteristics of set. Therefore in the first approximation it is
possible to consider, that D is fractal dimension
concerning homogeneous fractals in multifractal set.
Thus, to the basic
characteristics of a fractal belongs its dimension generalized or Hausdorff’s
D, correlated D2
and informative D1.
Hurst
's parameter
There are various ways of defining of fractal
dimensions to a number of which the so-called
R/S-way belongs, on the basis
of which
Hurst
's parameter is defined [3]. This parameter has wide application in
the analysis of time series owing to the remarkable stability. It
contains the minimal assumptions of studied system and can classify
time series. It can distinguish a random series from not random,
even if a random series is not Gaussian’s one (that is not
normally distributed).
For comparison of various
types of time series
Hurst
has entered a following ratio:
R/S=(a·N)Н,
where R/S – normalized scope from the saved up average, N
– number of supervision, and – some constant, Н
–
Hurst
's parameter.
There are three various
classifications for
Hurst
's parameter:
1) Н = 0.5.
Specifies a random series. Events are random and
uncorrelated. The present does not influence the future. Function of
density of probability can be a normal curve, however it is not
obligatory condition. The R/S-analysis can classify any series,
irrespective of what kind of distribution corresponds to it.
2) 0
≤ Н< 0.5. The given range corresponds to
antipersistent or ergodic series. Such type of system is
often called – «return to an average». If the system shows
"growth" during the previous period, most likely, in the
following period recession will begin. And on the contrary, if there
was a decrease close rise is probable. Stability of such
antipersistent behaviour depends on how much Н is
close to zero. Such series is more inconstant than random series as
it consists of frequent reversers of recession-rise.
3) 0.5
<Н <1.0. We
have persistent,
or trend
stable series. If
a series increases (decreases) during the previous period it is
probable, that it will keep this tendency for some time in the
future. More Н is closer to 0.5,
noisier a series is and less its trend is expressed. Persistent
series is the generalized Brown’s movement, or the
displaced casual wanderings. Force of this displacement depends on
how much Н is more than 0.5.
The fourth characteristic of a parameter of
Hurst
also exists, when Н>1.
In this case they speak about Levi statistics and about process (or
a time series) with fractal time, about time points of break of a
derivative. It means that there are independent jumps of amplitude
distributed on Levi in time, certain in the size of jump, and
growing together with it. The dispersion of an increment for the
given interval of time becomes final, the trajectory in phase space
keeps the kind, but new fractal object appears - time points of
break of a derivative.
If in double logarithmic
coordinates to find inclination R/S as function from N we
shall receive grade
N. This
grade is not connected with any assumptions concerning underlying
distribution though in the work [5] attempt of classification of
casual distributions on the basis of fractal scales is undertaken.
For very plenty of supervision N it is possible to
expect convergence of series to size Н=0.5 as the effect of memory
decreases up to that level when it becomes imperceptible. In other
words, in case of some supervision it is possible to expect, that
its properties become indistinguishable from properties of usual
Brown’s movement, or simple casual wandering as the effect of
memory dissipates.
Hurst
's parameter can be transformed in fractal
dimension D by means of the following formula [3-5]:
D=2-H
Fractal dimension of time
series, or the saved up changes at casual wandering, is 1.5. Fractal
dimension of a curve is 1, and fractal dimension of a geometrical
plane is 2. Thus, fractal dimension of casual wandering lays between
a curve and a plane. If Н = 0.5, D = 1.5. Both sizes
characterize independent casual system. The size 0.5 <Н 1 will
correspond to fractal dimension closer to a curve. It is persistent
time series, giving more smooth, less jagged line, rather than
casual wandering. Antipersistent size Н (0 <Н <0.5) gives
accordingly higher fractal dimension and more faltering line, than
casual wandering, and, hence, characterizes the system more subject
to changes.
For an example on рис.1 schedules of various
functions with
Hurst
's parameter (H), fractal dimension (D), correlation
dimension (D2) and correlated entropy
(D1) are presented.

Fig.1.
Fractal characteristics of functions
From resulted fractal characteristics it is easily
possible to track their change on change of the form of schedules of
functions. So, «noisier» functions by their fractal
characteristics differ from ideal. For example, functions W (x) and
G (x) which are described by identical expressions, but in W (x) a
noisy component is added and distributed evenly on an interval
[0-0.5]. If to consider, for example, sinusoidal functions G (x), G1
(x) and G2 (x) it can be noticed that with increase of the period of
function
Hurst
's parameter comes closer to Н=0.5.
It says about "approach" of function to a
straight line, i.e. the sinusoid "is extended" in a
straight line. Functions Е(х), Е1(х)
and Е2 (х)
are typical representatives of persistent series. Noise in Е2 (х)
is insignificant in comparison with the general trend stability,
therefore parameter Н is more than for functions Е
(x) and Е1 (x) where not only trend stability but also periodicity
are obviously expressed. And obviously various periods lightly
influence the change of parameter Н in comparison
with functions G (x), G1 (x) and G2 (x). Function A (x) represents
casual wandering argument that parameter Н displays
close to 0.5.
Thus, from the given
examples the interrelation between a kind of function (time series)
and
Hurst
's parameter which says about an opportunity of its use for
classification of functions (series) is visible. Meanwhile, there is
one more feature of definition of a parameter of
Hurst
. As it was marked above for its definition it is necessary to
approximate straight line R/S as function from N in double
logarithmic coordinates. But thus, as a rule, characteristic sites
of function log [R/S (N)] are not allocated and approximation
is carried out so-called «Averaging ». For example, for function
W (x) we have
Hurst
's precisely specifying periodicity (ant persistence) but nothing
speaking about a casual component which is not essential in
comparison with obviously expressed periodicity. For revealing such
prominent features of functions (time series) it is expedient to
carry out approximation not on "average" of function
log [R/S (N)], and on characteristic sites of this function.
For example, on fig. 2 the schedule of function log [R/S (N)]
(in figure the quantity of supervision or readout N is
designated through t), constructed on function W (x)
where two characteristic sites are confirmed to approximations.
Fig.2.
The schedule of function log [R/S (t)]
for W (x) and its approximations
Apparently
from figure two characteristic sites are well approximated by
corresponding straight lines which specify
Hurst
's parameters 0.12 and 0.505 that corresponds to periodicity and
noisiness of function W (x).
Fractal
characteristic of microgeometry of a superficial layer
For
studying fractal characteristics of microgeometry of a superficial
layer Talyrond traces were used, received after various kinds of
mechanical influence on a processable surface of steel preparations.
Fractal dimension of Talyrond traces was estimated under the
characteristics resulted above:
Hurst
's parameter, correlation dimension and correlated entropy.
Hurst
's parameter was defined as on "averaging" all arguments
(length of Talyrond traces) and on characteristic sites of function
log [R/S (t)].
To
more detailed researches Talyrond traces were exposed, received
after processing turning on various modes of submission and speed of
cutting.
On
Fig.3 schedules of dependences of
Hurst
’s parameter of a microstructure of the surface are presented and
calculated on "averaging" of function log [R/S (t)],
from submission and speed of cutting. Apparently from figure,
parameter Н is in a range 0.5...1 that specifies on
persistence of Talyrond trace. On some modes of processing casual
wandering a microstructure of a surface, i.e. Н is
marked®0.5.
Character of change of parameter Н specifies that the
microstructure of a surface received by turning has irregular
character, i.e. there is a tendency to its increase on a course of
processing. It hypothetically corresponds, for example, to functions
Е (x), Е1 (x) and Е2 (x) (fig.1) and insufficient rigidity of
system can indirectly specify display of such technology factors, as
deterioration of the tool, the vibrations.
Fig.3
Typical dependences of
Hurst
’s parameter of a microstructure of a surface on submission and
speed of cutting during turning.
On
Fig.4 typical dependences of
Hurst
’s parameter of a microstructure of a surface on arithmetic-mean
value of height of roughnesses are presented.
.
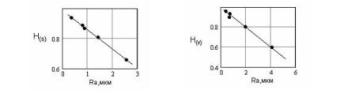
Fig.4
Typical dependences of
Hurst
’s parameter of a microstructure of a surface on arithmetic-mean
value of height of roughnesses (H (s) –
Hurst
's parameter from influence of submission, H (v) –
Hurst
's parameter from influence of speed of cutting)
But, as it was
specified above, functions log [R/S (t)]
on which
Hurst
's parameter is defined, have the characteristic sites specifying
both on periodicity, and on accident of a microstructure of a
surface (fig.5).

Fig. 5 Schedules of
functions log [R/S (t)] for
Talyrond traces received on various modes of processing at turning
steel 45
On
fig. 5 schedules log [R/S (t)], constructed for Talyrond
traces the surfaces processed on various modes at turning are
presented. Apparently from figure all functions have two
characteristic sites, displaying, as «noisy», and periodic
components of a microstructure of a surface. The angular factor of
approximating straight lines specifies
Hurst
's parameter. Analyzing schedules fig.5 it is possible to note, that
at increase in submission the periodic component of a microstructure
of a surface prevails, and at increase in speed of cutting –
noisy.
Research of
"scalability" of a microstructure of a surface which can
be estimated through dependence of height of a structure (Ra) from
quantity of "ledges-hollows" (NL) on
the fixed length of Talyrond trace
is of interest. On the basis of experimental researches schedules of
such dependences in double logarithmic coordinates (рис.6),
approximated are received by straight lines. The received
dependences are typical fractal, i.e. scale invariancy of a
microstructure is shown - with increase in height of roughnesses the
quantity of "ledges-hollows" decreases.
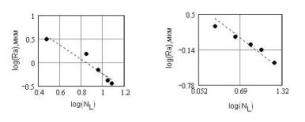
Fig. 6 Dependence of
arithmetic-mean value of height of roughnesses (Ra)
from quantity of "ledges-hollows" (NL) on the fixed length of Talyrond trace
at change of speed of cutting a() and sizes of submission (b) at
turning steel 45. Corresponding fractal dimensions »1.62
and »1.92.
On fig. 7
dependences log (NL/Ra) from the logarithm of sizes of
speed of cutting (a) and submissions (б)
are shown.

Fig. 7 Dependences
log(NL/Ra) on the logarithm of size
of speed of cutting (a) and submissions (b).
Corresponding
fractals dimensions »1.74 и »1.38.
On fig. 8
dependences log (S) and log (V) from the logarithm of
arithmetic-mean value of height of roughnesses are presented. As
show figures of 7 and 8 values of data in logarithmic scales settle
down practically on one straight line which says about their fractal
nature.
.
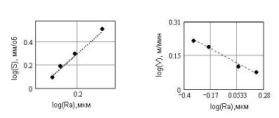
Fig.
8. Dependences log (S) and log (V) from log (Ra) at turning
processing steel 45. Corresponding fractal
dimensions »1.89
и »1.28.
Thus, display of
fractal properties of a microrelief of a surface says about
self-similarity of a microrelief. And self-similarity is shown not
only concerning geometrical scaling, but also concerning modes of
processing. Obviously, observable self-similarity is reached by
recurrence of the mechanism of the previous technological influence
on a surface, but in other "scale". In that case it is
necessary to find out this mechanism of "repeatability",
that, most likely, it is possible only from positions of
synergetrics and fractal materiology [6,7]. Besides it is possible
to assume, that fractal properties of a superficial layer display
processes of its self-organizing from the point of view of hierarchy
of fractal structures. Revealing of such hierarchies will allow
predicting and operating quality of a superficial layer of details
of machines.
Conclusions
On the basis of the
accomplished researches it is established:
1)
fractal properties of a microrelief of
the processed surfaces depend on conditions of processing of a
surface;
2)
The R/S-analysis of Talyrond
trace of surfaces allows to estimate character of a
microrelief from the point of view of periodicity (regularity) and
«noisiness», and also to define fractal dimension;
3)
the microrelief of a surface
(arithmetic-mean value, Ra) is self-similar or scale-invariant
concerning modes of processing (it is shown on an example of
turning);
4)
functional dependence between
parameter of roughness Ra and modes of processing is unequivocally
defined through fractal dimension.
The literature
1. Качество
машин: Справочник. В 2 т. Т.1 / А.Г.
Суслов, Э.Д. Браун, Н.А. Виткевич и др.
– М.: Машиностроение, 1995. – 256 с.
2. А.А.
Потапов, В.В. Булавкин, В.А. Герман и др.
Исследование микрорельефа
обработанных поверхностей с помощью
методов фрактальных сигнатур. //
Журнал технической физики, 2005, том 75,
вып. 5. – С. 28-45.
3.
Федер Е. Фракталы. Пер. с англ. – М.:
Мир, 1991. – 254 с.
4.
Божокин С.В., Паршин Д.А. Фракталы и
мультифракталы. – Ижевск: НИЦ «Регулярная
и хаотическая динамика», 2001. – 128 с.
5.
Ю.Н. Кликушин. Фрактальная
шкала для измерения формы
распределений вероятности // Журнал
радиоэлектроники № 3, 2000. – С. 15-18.
6.
Хакен Г. Синергетика. - М.: Мир, 1980. – 400с.
7.
Иванова
В.С., Баланкин А.С., Бунин И.Ж. и др.
Синергетика и фракталы
в материаловедении.
- М.: Наука, 1994. – 383 с.