Abstract
One of basic telecommunication system tendency is its digitization. In
any digital system one of the most important parameter is sampling.
Quality of system work depends from accuracy of this parameter.
Undesirable phase and/or frequency random discrecity period departures
are calling jitter. It may arise in the issue of master oscillator
instability, line parameter changing in time and different frequency
component propagation speed of same signals [1]. Jitter is one of main
problems digital electronics device development, specifically digital
interfaces. Not enough careful jitter calculation can lead to its
accumulation while signal forwarding in digital channel and, at the end,
to device nonoperability.
In telecommunication sphere jitter and its consequences prevention
passes by the instrumentality of buffer storage, PLL devices, special
linear code use, and clock synchronization dedicated line creation.
Basically this solution presupposes hardware support. But in certain
cases postprocessing and signal extraction by software tools can take
place. This work is about jitter minimization devices progress on
discretization signal phase. Per se, jitter deletion task adds up to
restoration of stochastically sampled signal characteristics. [2]
One of the new, not enough explored now, restoration of stochastically
sampled signal characteristics approach is method which uses integral
transformations [3]. This method conception can be shown as a scheme
(pic. 1).
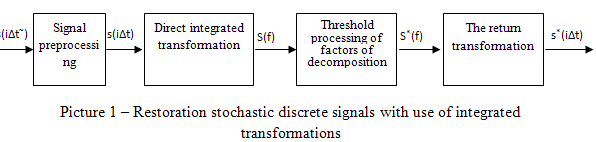
According to this structure irregularly sampled signal s(iΔt~)
conversion to signal with fixed lead s(iΔt) takes place on the fist
step. Then direct integral transform takes place, which convert signal
from time representation to frequency. The alias components have
broadband noise-type spectrum in stochastic sampling [5], and according
to this they can be minimized by expansion coefficient thresholding S
(f). After this inversion is performed, i.e. a signal s*(iΔt)
is generated that has constant sampling step with reduced alias
component levels. Fourier discrete transformations (DFT) may be used as
integral transforms in this structure or its version – discrete cosines
transformation (DCT) and discrete sinus transformation (DST) [4].
But this structure isn’t unique because it can be used only for
stationary signals restoration that have line spectrum, as Fourier
transformations make signal characteristics averaging-out on whole
cultivated signal part, as follows local features may be lost.
Using of wavelet signal decomposition as integral transform may help to
avoid this problem. It doesn’t have such defects [4]. This expansion is
given by [8]
(1)
where
ψ(t)
– parent function (parent wavelet);
а
– the factor setting plotting scale of wavelet (it is found to
frequency);
b
– the factor setting position of wavelet (it is proportional to time).
On practice discrete wavelet signal transformation is used more often,
as it may be realized using LF and HF filters set detailed expansion
coefficients arrive as a result of pass though LF-Filter, and
approximations coefficients are a result of HF filtering .
Advantages of this signal decomposition method are:
·
Features of all local signals, which stores all transferred information,
are taked into account.
·
This expansion is simple in realization and doesn’t make additional
superfluity in initial data.
· Back transformation also is realized by using filters.[9]
In this work a new way of jitter that occurred on sampling phase
prevention was offered. This method is based on using of
пороговой
treatment of wavelet signal decomposition. It also may be used for lost
digital signal sample reconstruction. This method using in
telecommunication systems can help to increase information transferring
reliability.
References:
1. Джулиан Данн. Джиттер. Теория. Audio Precision.
http://www.ixbt.com/proaudio/jitter-theory-part3.shtml
2. Сторожук Н.Л., Белоруков В.А., Щитников В.И., Еще раз о джиттере.
Метрология и измерительная техника в связи 2 2006г. 49-52с.
3. Дегтяренко И.В., Афанасьев Д.Н. Алгоритм восстановления стохастически
дискретизированых сигналов, Наукові праці ДонНТУ. Серія «Обчислювальна
техніка та автоматизація. Випуск 15.- Донецьк: ДонНТУ, 2008- 36-41с.
4. Bilinskis I. Digital alias-free signal processing. – Willie, 2007. –
430 р..
5. Дмитриев В.И. Прикладная теория информации: Учебник для вузов.- М.:
ВШ, 1989г.320 с.
6. Beutler F. Alias –
free randomly timed sampling of stochastic
processes. — IEEE Trans. Inf. Theory, Vol. IT- 16, No. 2, 1970. pp.
147–152 с.
7. Корн Г., Корн Е. Справочник по математике для научных работников
и инженеров. – М.: Наука, 1973г,
831с.
8.
Дьяконов В.П. Вейвлеты. От теории к практике. – М.: СОЛОН-Р, - 2002. –
448 с.
9. Астафьева Н.М. Вейвлет-анализ: Основы теории и примеры применения. –
Успехи физических наук, 1996, т.166, № 11, 1145-1170с.
10. Левкович-Маслюк Л, Переберин А. Введение в вейвлет-анализ: Учебный
курс. – Москва, ГрафиКон’99, 1999. 120c.
|