Abstract
Introduction
For most modern enterprises a timely and reasonable management an issue and realization of products becomes one of terms of survival and successful functioning. Thus providing of efficiency of such management requires ability to expect the credible future state of enterprise and environment which it exists in, in time to warn possible failures and derangements in-process. It is arrived at by prognostication of the both planned and practical work of enterprise on all directions his activity, and in particular, in area of prognostication of sale products.
1 Theme urgency
Planning of production activity is on an enterprise the important function of management of operations. The public functions of management directly behave to the planned activity of enterprises, and they in same queue serve as their basis. Actuality of this theme in modern terms is determined that level of prognostication of processes of community development it is the condition of the effective planning and management of operations.
2 Objectives and tasks of the study
The main feature of enterprise is that it produces products in accordance with often changing orders. Term of execution of every order, both on an amount and on a nomenclature, calculated by hours and always less than a day. The issue of products is closely related to the sale, because mine-out, but not realized in time products are recurrent wastes of production. The both prepared and uncompleted products eliminate possibility of the protracted storage. In addition, a production process is continuous, and interference with him, from point of operative management, it is practically impossible. That regulative influences can be carried out only before start of party in a production.
The indicated features substantially influence on organization and planning of economic activity of enterprises of this industry. Coming from said, it is very important to do the correct choice of method of prognostication. In this article the questions of method of prognostication of activity of enterprise are considered on the whole and to sale activity in particular.
3 Review of research-and-developments
Presently the statistical methods of prognostication took the visible place in world and national practice. The review of sources of methods, in-use for authentication of models of temporal rows is given in next sections.
3.1 Global review
Bases of the use of information about a cross-correlation function (or functions) unidimensional and multidimensional temporal rows considered the English scientists Boxing of Dzh. and G.Dzhenkinsom in a book Analysis of temporal rows, prognosis and management
[1]. The special attention is spared non-stationary temporal rows, to containing either stationary increases or periodic. Chapters, containing basic information from the cross-correlation theory of casual processes, entered in the first issue, choice of model, evaluation of its parameters and verification of model, and also models for seasonal temporal rows.
In the article of candidate of economic sciences, item teacher of department of economy and enterprise Nizhegorodskogo an architectural-build university of Koshechkin S. Algorithm of prognostication of volume of sales
is presented one of possible algorithms of construction of prognosis of volume of realization for products with seasonal character of sales [2].
The row of reasons edited Semenycheva v.k. and Pavlova V.D. from the Samara municipal institute of management examines the new going near a design and prognostication of temporal rows on the basis of self-reactance models [3].
3.2 National review
The Train aid Analysis of temporal rows in an economy: contains the practical worker of application
edited a teacher DONNU Khristianovskogo v.v. substantive theoretical provisions for the analysis of temporal rows and drafting with their help of prognosis [4].
In-process there is Exposure of tendencies of temporal rows (manytrends model)
k.t.n. Smirnova A.V. and Mikhaylova s.v. the Donetsk state institute of artificial intelligence the hindcasting of economic descriptions is considered [5].
3.3 A local review
In-process master's degree of DONNTU Ruff Tat'yany Igor is produced research of efficiency of the computer point-of-sale systems, using a cross-correlation analysis.
4 Prediction of production and sales
The efficiency of enterprise management requires the ability to anticipate the likely future state of the enterprise and the environment in which it exists, in time to prevent possible malfunctions and failures in their work. This is achieved by projecting both planned and practical work of the enterprise in all directions of its activity, and in particular in forecasting sales.
4.1 Determining the nature of seasonal fluctuations
The first step in the implementation of any approach to forecasting performance, the changes are subject to periodic seasonal fluctuations, is to determine the nature of seasonality. This is due to the fact that the value of the projected figures in this case are formed under the influence of three components - trend, seasonal and random components, and depending on the relationship between the building blocks of a time series of indicators can be described by models with additive or multiplicative seasonal nature. In this additive model is the sum of the components (1), and multiplicative - in the form of their works (2):
(1) Pt = T + S + E,
(2) Pt = T + S + E, where the Pt - levels of time-series, T - the trend component, S - the seasonal component, E - the random component.
Thus, for additive seasonal fluctuations characteristic to be measured in absolute values and reflected in the statistical model in terms of form, and for the multiplicative fluctuations - in relative terms in the model and presented in the form factors [6]. In Figure 1 graphs product sales, compiled from the above models predict.
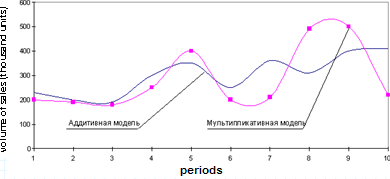
Figure 1 – The additive and multiplicative models predict
A distinctive feature of the additive model is that the amplitude of seasonal fluctuations, reflecting the deviation from the trend, or average, is approximately constant, unchanging in time. When the multiplicative nature of the seasonality of the oscillation amplitude varies in time proportional to the average level and trend of the series.
4.2 Analysis and modeling of the main trends in the predicted values
Search for the optimal expression of the trend component and its mathematical description are one of the most difficult and responsible phases of seasonal forecasting, on which depends largely on the accuracy and reliability of the final predictions. To investigate the nature of the general trend of changes in the levels of the series and its description method of calculation used moving averages and analytical alignment.
The essence of the analytical smoothing is to replace the actual values of the calculated index, calculated on the basis of theoretical models of growth curves that represent the different functions of time. With this analytical approach is an important element of choice of the optimal shape of the curve to level, after which the statistical evaluation of the unknown coefficients of the model, and then aligned with the values determined by a number of levels by substituting the corresponding values of the time parameter in the equation of the trend found by the model.
The method of calculating the moving average refers to the algorithmic approach, which does not require a description of non-random component with a single function, and provides only an algorithm to calculate it at any time. In this method, the actual data are replaced with the mean values calculated for sequentially moving the enlarged intervals, covering a number of levels of time series. By the indisputable advantages of moving averages is that they are easily calculated, explained, interpreted, and also allow smooth both random and periodic variations, and identify the existing trend in the development process under investigation.
It is proposed to use the approach of applying the method of moving averages for the preliminary calculation of the trend component in the subsequent quantification and elimination of seasonal effects and random effects modeling and final trend is based on analytical smoothing off-season series.
As an optimality criterion for choosing the form of the curve was used to align the coefficient of determination, showing the extent to which the theoretical model of the actual data. The coefficient is always between 0 and 1, and the closer it is to unity, the better the model approximates the original data.
To further define the optimal order of the polynomial approximating method of successive differences.
After statistical evaluation of the unknown coefficients of the model by least squares, we obtain an analytical equation of the trend. Substituting in the equation is the corresponding value of the time parameter t, we determine the values are aligned.
In line with this approach for the preliminary elimination of seasonal fluctuations was carried out the calculation of moving averages [7].
4.3 Algorithm for calculating the seasonal component
A mandatory part of any seasonal forecasting techniques is the quantification of the seasonal component, which depends on the nature of seasonality. Algorithm for calculating the seasonal component in the additive model adopted for the prediction consists of the following steps:
- To assess the cumulative effect of seasonality and random deviations are calculated from the values actually existing levels of smoothed series;
- For the elimination of the influence of random factors are determined by preliminary estimates of the seasonal component by averaging the values for like months;
- Adjustments to the initial values of the seasonal component, due to the fact that the net effect of seasonality on the dynamics of values should be neutral. In this regard, for the additive model, the sum of the seasonal component for a complete seasonal cycle should be zero.
For the multiplicative form of seasonal changes the contents of the first and third stages of the algorithm. First, instead of the absolute deviations calculated by indexes of seasonality ratio actually existing values to the corresponding levels of the smoothed series.
After obtaining preliminary estimates of seasonality in the second step is an adjustment. Paid off seasonal variations in the multiplicative form is expressed in the fact that the arithmetic mean of the values of seasonality indexes for a full seasonal cycle should be equal to 1 [8].
4.4 Modeling the dynamics of the original series
A distinctive feature of the method for predicting seasonal variations is that after the expansion of the original time series into components by the procedure of elimination of the influence of seasonal and random factors, and is the final assessment of the trend component. It is important to note that seasonal adjustment, aimed at cleansing the original time series of seasonal effects, in general contributed to a significant increase in the reliability of the approximation of all types of trend models, as evidenced by higher estimates for the coefficient of determination off-season series. However, the maximum values of this criterion is still consistent with the curves of polynomial type is why they were chosen for use as trend models. To determine the optimal order of approximating polynomial again used the method of successive differences, according to which it was necessary to choose a quadratic polynomial. In Figure 2 shows a graphical representation of a polynomial and a linear trend.
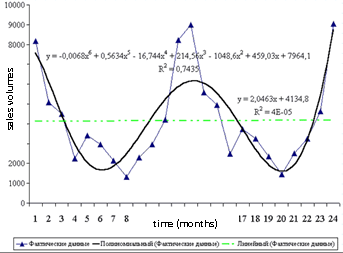
Figure 2 – Comparative analysis of polynomial and linear trend
Construction of trend-seasonal model with any approach to the prediction of complete modeling of the dynamics of the original series, which, if adopted by the seasonal nature of the additive is obtained by summing the estimates of trend and seasonal components.
To calculate the predicted values, you must first determine the levels of the trend for the corresponding values of the time parameter, which must be added the earlier estimate of the seasonal component.
To quantify the relative accuracy of the trend-seasonal model is calculated using the formula mean error of approximation.
4.5 Construction of the final predictions
Prediction based solely on the construction of trend-seasonal patterns, which are based on the assumption of unchanged trends and seasonal effects in time, may not contribute to obtaining reliable results. In this regard, the construction of the final forecast to account for potential changes in economic trends that have occurred in the past, and the possible emergence of new trends in the market it is advisable to use the method of exponential smoothing. This method allows to calculate the forecast, taking into account the values obtained by the theoretical model and actual values in the previous time period. In Figure 3 shows the final model for prediction of sales.
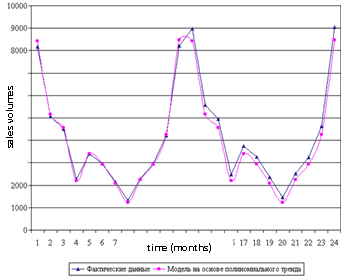
Figure 3 – Model prediction of volume of sales
An important issue that arise when using the method of exponential smoothing is the choice of the values of the smoothing constant, which depends on the period of anticipation and quickness of the forecast changes in market conditions. In the case of rapid, short-term forecasts, and minor changes in the market recommend that you choose is close to 1. In Figure 4 shows the basic steps of constructing the forecast in graphical form:
- sales forecast for the previous 2 years;
- trend with a period of 12 months;
- sum of trends with periods of 6 and 12 months;
- sum of trends with periods of 12, 6, 4 and 3 months;
- sum of periodic and linear trends;
- sum of trend and seasonal component.
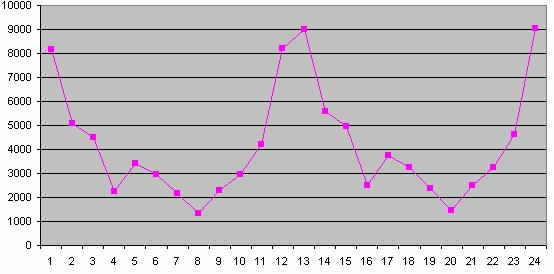
Figure 4 – Phase-change trend analysis (animation: 6 frames, 10 reps, 153 KB)
Conclusion
The chosen method of predicting seasonal variations based on a combination of moving averages and analytical alignment helps to ensure a more accurate and reliable forecasts and can be recommended for use in the practice of companies. In addition, individual elements of the methodology used in adapted form can be used to predict a number of conjugated economic indicators (sales, value of turnover), characterized by seasonal changes.
The advantages of the proposed method include its simplicity, accessibility, the ability to develop operational and short-term predictions with high accuracy. However, the considered prediction algorithm is based on the immutability of the time the main trends and seasonal effects, which do not always reflect the real dynamics. In this regard, the development of reliable and accurate forecasts should undertake a systematic analysis of changes in the market and if necessary, make appropriate adjustments and refinements to the model used for the purpose of its adaptation to new conditions. Thus, the implementation of this method of forecasting should not be approached formally, because of its success can be achieved only if the competent combination of deep meaningful economic-theoretical analysis of the professional knowledge of the specifics of the market.
This master's work is not completed yet. Final completion: December 2012. The full text of the work and materials on the topic can be obtained from the author or his head after this date.
References
- Алексеева М.М. Планирование деятельности фирмы: Учебно-методическое пособие. М.: Финансы и статистика, 1997.
- Андрейчиков А.В., Андрейчикова О.Н. Анализ, синтез, планирование решений в экономике. — М.: Финансы и статистика, 2000. — 368 с.
- Анискин Ю.П. Внутрифирменное планирование: Учебное пособие. М. МГИЭТ (ТУ), 1994.
- Ансофф И. Стратегическое управление М. Экономика 1989
- Баззел Р.Д. и др. Информация и риск в маркетинге. — М.: Финстатинформ, 1993.
- Беляевский И.К. Маркетинговое исследование: информация, анализ, прогноз. — М.: Финансы и статистика, 2001.
- Березин И.С. Маркетинг и исследования рынков. — М.: Русская деловая литература, 1999.
- Бестужев-Лада И.В. Прогнозное обоснование социальных нововведений. – М.: Наука, 1993.
- Бешелев С.Д., Гурвич Ф.Г. Экспертные оценки в принятии пла-новых решений. М.: Экономика, 1976.
- Бокс Дж., Дженкинс Г. Анализ временных рядов, прогноз и управление: Пер. с англ. // Под ред. В.Ф. Писаренко. – М.: Мир, 1974, кн. 2. – 197 с.
- Бондаренко А.В. Доработка алгоритма прогнозирования объема продаж / А.В. Бондаренко Режим доступа: http://www.cfin.ru/finanalysis/math/add_to_kosh.shtml/
- Бухалков М.И. Внутрифирменное планирование М.: Инфра-М, 2001.
- Веревченко А. П., и др. Информационные ресурсы для принятия решений Издательства: Деловая Книга, Академический проект; 560 стр., 2002 г.
- Владимирова Л. П. Прогнозирование и планирование в условиях рынка. Учебное пособие. — М.: Дашков и К., 2000. — 308 с.
- Волокитин А. В., и др. Средства информатизации государственных организаций и коммерческих фирм. Справочное пособие Издательство: ФИОРД-ИНФО 272 стр., 2002 г.
- Гаскаров Д. В. Интеллектуальные информационные системы Издательство: Высшая школа, 432 стр., 2003 г.
- Глазьев С.Ю. Теория долгосрочного технико-экономического развития. М., 1993
- Глинский В.В., Ионин В.Г. Статистический анализ. — М.: Филинъ, 1998. — 264 с.
- Голубков Е.П. Маркетинговые исследования: теория, методо-логия и практика. М.: Финпресс, 1998.
- Дж.Гласс, Дж.Стенли. Статистические методы в прогнози-ровании. М.: Прогресс, 1976.
- Дмитриев С. Уязвимость прогнозной модели продаж Кошечкина / С. Дмитриев Режим доступа: http://www.marketing.spb.ru/lib-mm/sales/brand_portfolio.htm/
- Дуброва Т.А. Статистические методы прогнозирования. — М.: ЮНИТИ-ДАНА, 2003. — 206 с.
- Евланов Л.Г., Кутузов В.А. Экспертные оценки в управлении. М.: Экономика, 1978.
- Егоров В.В., Парсаданов Г.А. Прогнозирование национальной экономики: Учеб. пособие. – М.: ИНФРА – М, 2001.
- Елисеева И.И., Юзбашев М.М. Общая теория статистики / Под ред. И.И. Елисеевой. М.: Финансы и статистика, 1995.
- Ефимова М.Р., Рябцев В.М. Общая теория статистики. — М.: Финансы и статистика, 1991.
- Загинайло И.В. Периодические линии тренда в прогнозировании объемов продаж / И.В. Загинайло // Режим доступа: ttp://www.cfin.ru/finanalysis/math/add_to_koshbond.shtml/
- Ильин А.И. Управление предприятием М.: Высшая школа 1997.
- Кошечкин С.А. Алгоритм прогнозирования объема продаж в MS Excel // Маркетинг в России и за рубежом. — 2001. — №5. Режим доступа: http://www.cfin.ru/finanalysis/sales_forecast.shtml
- Коренев А. Прогноз продаж статистическим методом / А. Коренев Режим доступа: http://www.cfin.ru/finanalysis/math/statistical_method.shtml/
- Кофман А., Фор Р. Займемся исследованием операции. М.: Мир, 2002
- Кунц Г., О’ Доннел С. Управление. Системный и ситуационный анализ управленческих функций. Пер. с англ.: В 2 т. Т. 2 / Общая ред. акад. Д.М. Гвишиани. М.: Прогресс, 1981.
- Леонтьев В. Экономические эссе. Теория, исследования, факты и политика / Пер. с англ. М.: Политиздат, 1990.
- Лисичкин В.А. Отраслевое научно-техническое прогнозирование. – М.: Экономика, 1971.
- Литвак Б.Г. Экспертные оценки и принятие решений. — М.: Патент, 1996.
- Лобанова Е. Прогнозирование с учетом экономического роста // Экономические науки. — 1992. — № 1.
- Мазманова Б.Г. Основы теории и практики прогнозирования: учебное пособие. Екатеринбург: изд. ИПК УГТУ, 1998.
- Мамиконов А.Г. Принятие решений и информация. М.: Наука, 2002
- Математика и кибернетика в экономике. М.: Экономика, 1975
- Мескон М.Х., Альберт М., Хедоури Ф. Основы менеджмента. М.: Дело, 1993.
- Новоселов Ю.А. Социально-экономическое прогнозирование. Ч.1– Новосибирск: СибУПК, 2000.
- Общая теория статистики / Под ред. Спирина А.А., Башиной О.Э. — М.: Финансы и статистика, 1997. — 296 с.
- Октябрьский П.Я. Статистика. — СПб.: СПбГУ, 1999. — 223 с. 7. Теория статистики / Под ред. Г.Л. Громыко. — М.: ИНФРА-М, 2000. — 414 с.
- Отнес Р., Эноксон Л. Прикладной анализ временных рядов. - М.: Мир, 1982.
- Петров Л.Н. Стратегическое планирование развития предприятия С. Питербург 1993.
- Перегудов Ф.И., Тарасенко Ф.П. Введение в системный анализ. М.: Высшая школа, 2001
- Прогнозирование и планирование экономики. — Экоперспектива, 2000. — 432 с.
- Рыночная экономика: Учебник. Т. 1. Теория рыночной экономики. Часть 1. Микроэкономика / Под ред. В.Ф. Максимова — М.: Соминтэк, 1992.
- Синюк В.Г., Шевырев А.В. Использование информационно-аналитических технологий при принятии управленческих решений Издательство: ДМК Пресс; 160 стр., 2003 г.
- Смирнов А.В., Михайлов С.В. Выявление тенденций временных рядов (многотрендовая модель). — М.: Финансы и статистика, 1995.
- Статистический словарь / Под ред. М.А. Королева — М.: Финансы и статистика, 1989.
- Статистическое моделирование и прогнозирование: Учебное пособие / Под ред. Семёнычева В.К., Павлова В.Д. — М.: Финансы и статистика, 1990.
- Теин А. Г. Земля Информатика. Екатеринбург: Издательство Уральского университета. Издательство Дома учителя, 2002
- Теория статистики / Под ред. Р.А. Шмойловой. — М.: Финансы и статистика, 2000. — 414 с.
- Уткин В. Б., Балдин К. В. Информационные системы в экономике Издательство: Финансы и статистика , 288 стр., 2004 г.
- Христиановский В.В. Анализ временных рядов в экономике: практика применения. М.: Наука, 1993.
- Четыркин Е.М. Статистические методы прогнозирования. 2-е изд., перераб. И доп. М.: Статистика, 1997.
- Экономика предприятия (фирмы) / Под ред. Волкова О.И., Девяткина О.В. — М.: ИНФРА-М, 2003. — 601 с.
- Юзбашев М.М., Манелля А.И. Статистический анализ тенденций и колеблемости. — М.: Финансы и статистика, 1983.
- Янч Эрих. Прогнозирование научно-технического прогресса. Пер. с англ. М.: Прогресс, 1974.
- Aaker, David A. and Day George S. Marketing Research. — 4th ed. — NewYork: John Wiley and Sons, 1990. — Chapter 22 «Forecasting».
- Dalrymple, D.J. Sales forecasting practices // International Journal of Forecasting. — 1987. — Vol. 3.
- Kress G.J., Shyder J. Forecasting and Market Analysis Techniques: A Practical Approach. — Hardcover, 1994.
- Schnaars, S.P. The use of multiple scenarios in sales forecasting // The International Journal of Forecasting. — 1987. — Vol. 3.
- Waddell D., Sohal A. Forecasting: The Key to Managerial Decision Making // Management Decision. — 1994. — Vol 32, Issue 1.
- Wheelwright, S. and Makridakis, S. Forecasting Methods for Management. — 4th ed. — John Wiley & Sons, Canada, 1985.